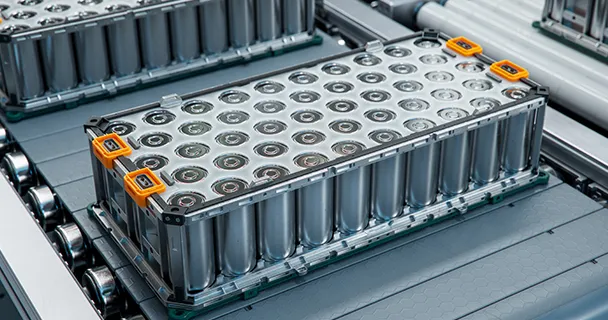
Electric Vehicles Are Embracing New Trends in 2024 - January 26, 2024
The automotive world is rapidly evolving as today’s cars gain access to technology that was impossible just a decade ago. In China, two automakers are rolling out electric passenger vehicles powered by sodium-ion batteries. The move comes in response to fluctuations in lithium prices that put pressure on the EV market. Â
Meanwhile, AI remains the biggest trend in all technology. Volkswagen is partnering with a startup called Cerence to bring ChatGPT features into its vehicle lineup. Drivers will be able to interact with the AI assistant for both simple and complex queries while on the road. Â
Two Chinese Automakers Launch EVs With Sodium-Ion Batteries
With the popularity of electric vehicles (EVs) reaching new heights, the batteries that power them are coming under the microscope. Though it may seem trivial to those on the outside, the type of battery under the hood is a significant factor in determining both driving range and the total cost of the vehicle. In China, the world’s largest EV market, sodium-ion-based batteries are making their debut to upset the reign of lithium-ion batteries. Â
Recent reports highlight two new EVs powered by sodium-ion batteries arriving on the market. The first comes from JMEV, an EV offshoot of the Chinese Jiangling Motors Group. It boasts a sodium-ion battery manufactured by China’s Farasis Energy with an energy density between 140Wh/kg and 160Wh/kg. For comparison, most lithium-ion batteries feature an energy density between 260Wh/kg and 270Wh/kg. Â
Notably, the JMEV vehicle has a driving range of 251 kilometers (about 156 miles). This range makes it an acceptable choice for daily commuting or cross-city travel, though it won’t be winning any awards for its longevity. Compared to leading lithium-ion EVs, which boast ranges over 300 miles per charge, this sodium-ion EV is underwhelming. Â
However, it’s an exciting new addition to the market given the potential for growth and savings in the sodium-ion battery segment. Compared to their lithium-based cousins, sodium-ion batteries feature superior discharge capacity retention. The JMEV model’s is a respectable 91%. Â
Meanwhile, the second Chinese EV to feature a sodium-ion battery comes from Yiwei, a brand belonging to the JAC Group. The battery itself is made by Hina battery and gives the vehicle a range of 252 kilometers. Â
Sodium-ion battery technology is expected to improve significantly over the next few years. Farasis claims the energy density of its batteries will increase to between 180Wh/kg and 200Wh/kg by 2026. It claims this will make the battery more relevant for a broader range of applications, including energy storage and battery swapping. Â
Another factor to consider is the variable price of lithium and its ripple effect on the EV market. In 2022, sharp price hikes spurred manufacturers to devote more resources to sodium-ion battery research and production. Unsurprisingly, Farasis and Hina aren’t the only firms making a push into the market. China’s CATL and Eve Energy also launched their sodium-ion technology in response to the rising prices of lithium. Â
Given that China imports roughly 70% of its lithium, price swings are a significant factor for the industry to consider. Since experts believe sodium-ion battery prices will drop as they enter mass production, these could be a viable alternative for the industry. Â
However, experts warn that slumping lithium prices will quell interest in sodium-ion battery research and adoption. How this trend plays out in 2024 will largely depend on lithium prices—not the booming demand for EVs in China and beyond.
Volkswagen Partners with Cerence to Bring ChatGPT Features to Its Vehicles
Just when it seemed like ChatGPT was everywhere, the artificial intelligence (AI) chatbot is expanding its presence into cars. Thanks to a partnership between Volkswagen and Cerence, an AI-focused tech startup, ChatGPT will soon be integrated into the carmaker’s Ida voice assistant. The news, announced during CES 2024, arrives with a mixed reception from drivers. Â
For those wary of integrating AI with their vehicle’s native software, there is a bright side. The car’s Ida assistant will still handle tasks like voice-powered navigation and climate control changes. However, Cerence’s Chat Pro software will soon be able to handle more complex queries through the cloud. Â
Drivers can pose natural language, open-ended queries like “Find me a good burger restaurant nearby.” Cerence’s software then processes the request and sends an answer back through the Ida assistant or the built-in navigation system. Â
As one might expect from ChatGPT, the integration can also handle more nuanced queries. A recent Volkswagen ad showed Ewan McGregor using “The Force” (the Cerence ChatGPT integration) to inquire about wearing a kilt to an upcoming event. The software supplied a detailed response advising the actor on the appropriateness of wearing kilts to formal gatherings. The ChatGPT integration will also be able to offer interactions like providing trivia questions for long road trips or reading a bedtime story for a child in the back seat. Â
According to Cerence, the ChatGPT integration gives drivers access to “fun and conversational chitchat” at the push of a button or voice command prompt. Looking ahead, Volkswagen and Cerence plan to continue their collaboration to add more ChatGPT features to the Ida voice assistant. Â
Drivers can expect ChatGPT functionality to arrive in the second quarter of 2024. The update will roll out over the air, and Volkswagen claims it will be “seamless.” An extensive lineup of Volkswagen vehicles, including both electric and gas-powered models, will support the integration. ID 3, ID 4, ID 5, ID 7, Tiguan, Passat, and Golf will be the first to receive the update. Â
Of course, while this is an exciting update for Volkswagen fans, there is the question of whether cars need access to ChatGPT’s features. The last thing the roads need is more distracted drivers. While drivers can interact with ChatGPT hands-free, it’s easy to envision someone getting a bit too involved with answering trivia questions behind the wheel and losing focus on the road. At this time, it’s unclear whether Volkswagen and Cerence have a plan in place to address these safety concerns. Â
Despite this, similar features will likely arrive in vehicles of other makes in the days ahead. ChatGPT is currently one of the hottest topics of conversation in the tech world, and automakers are likely scrambling to catch up with Volkswagen and add it to their vehicles.
‍
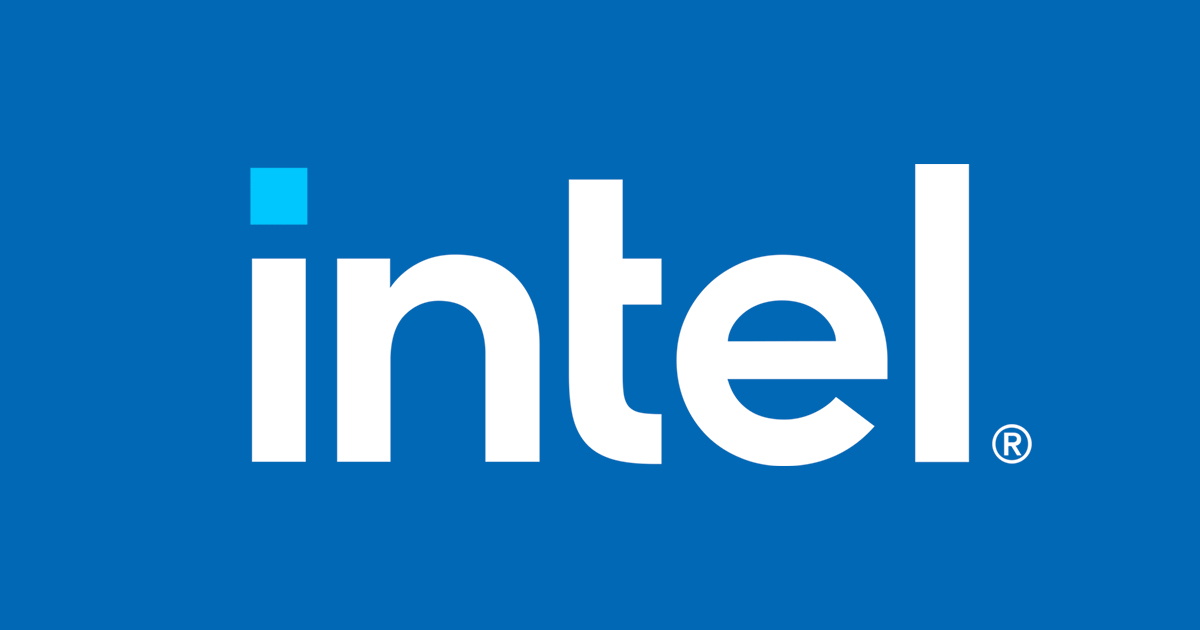
AI Preparing for Another Busy Year - January 9, 2024
‍The buzzing excitement of a new year always brings plenty of change and interesting perspectives for the chip sector. From new product releases to outlooks on the latest trends, manufacturers and buyers are preparing for a busy year. AI promises to be a key technology in 2024, and Intel’s latest Xeon processor, which boasts AI acceleration in every core, is a testament to it. Â
Meanwhile, memory prices are continuing their surge from the end of 2023 thanks to sustained production cuts from the world’s biggest manufacturers. As buyers scramble to secure inventory amid fresh demand from China’s smartphone market, the price trend for memory chips looks bright. Â
Intel’s Latest Xeon Processors Feature AI Acceleration in All 64 Cores
Computing headlines in 2023 were dominated by artificial intelligence (AI). Experts believe the same will be true in 2024 as tech leaders around the globe further expand the integration of AI with traditional hardware and software. Intel’s forthcoming 5‍th Gen Xeon processors are no exception. The new chips are built with AI acceleration in each of their 64 cores to improve both efficiency and performance.  The chipmaker’s latest breakthrough doesn’t stand alone. Adding AI to traditional computing hardware
‍ is one of the hottest trends in chipmaking. It promises to play a central role in the industry over the coming years as more ways for end users to harness the power of AI on their devices are introduced. Â
However, experts have also warned that increased usage of AI puts a hefty strain on the world’s power supply. Many fear the technology could consume more power than entire countries as its popularity skyrockets. One way to offset this power-hungry tech is through more efficient chips—a feature Intel highlighted during the 5th Gen Xeon unveiling. Â
Intel says the new chips will enable a 36% higher average performance per watt across workloads. This is a significant energy reduction and will surely entice buyers who closely monitor their cost of ownership. Over the long run, systems using Intel’s newest Xeon chips will use far less energy than those running on older processors. Â
Despite being more efficient, the new Xeon processors also deliver a notable performance increase of 21%, on average, compared to the previous generation. For AI work, such as large-language model (LLM) training, the chips boast a 23% generational improvement, according to Intel executive vice president and head of the Data Center and AI Group, Sandra Rivera. For LLMs under 20 billion parameters, the new Xeon chip delivers less than 100-millisecond latency, enabling faster performance for model training and generative AI projects. Â
Per Intel’s press release announcing the new chip, including AI acceleration in every core will “address demanding end-to-end AI workloads before customers need to add discrete accelerators.” Â
Indeed, upgradeability is another key selling point for the 5th Gen Xeon processors. The chips are pin and software compatible with the previous generation.
Rivera says, “As a result of our long-standing work with customers, partners and the developer ecosystem, we’re launching 5th Gen Xeon on a proven foundation that will enable rapid adoption and scale at lower TCO.” Â
The first equipment featuring Intel’s new Xeon chips will arrive in the first quarter of 2024. Buyers can expect offerings from leading OEMs, including Lenovo, Cisco, Dell, HPE, IEIT Systems, Super Micro Computer, and more. Â
As AI adoption continues, expect to see more acceleration built into next-gen silicon. While it’s unclear how far the AI trend will advance, the technology isn’t going away anytime soon. By contrast, it seems AI will play a pivotal role in the future of computing and humanity. Don’t be fooled by the feeling that AI is already everywhere. According to Intel CEO Pat Gelsinger, the technology is likely “underestimated.” Â
With that perspective leading the way, expect Intel to focus more intensely on AI chips in the years ahead. The 5th Gen Xeon processor is a meaningful step in this direction, and more will surely follow. Â
‍NAND Flash Wafers See 25% Surge Amid Sustained Production Cuts
Aggressive production cuts are positively impacting memory chip prices‍ practically across the board, according to new data from TrendForce and insights from industry experts. While the likes of Samsung, SK Hynix, and Micron have repeatedly slashed production—particularly of NAND Flash modules—buyers have burned through their inventories and are now rushing to secure more. Â
As the year begins, peak season demand from the holiday production cycle is waning, but overall demand for memory chips hasn’t slowed down. Experts point to persistent production cuts as the primary driver creating a supply and demand imbalance. As a result, memory chip makers can dramatically raise prices. Â
In November alone, NAND Flash wafers saw their price skyrocket by 25%, according to data from TrendForce. This rise closely followed Samsung’s decision to slash its total production capacity by 50% in September. While the rise is painful for buyers looking to source new memory components, experts have gained confidence in the pricing of those chips. Â
Interestingly, production cuts aren’t the only factor behind the price increase. Experts point to the surging Chinese smartphone market as another noteworthy factor. Led by Huawei and its Mate 60 series, smartphone manufacturers in China are working to regain market share after falling behind thanks to chip export restrictions put in place by the U.S. and its allies. As the Chinese chip sector seeks to reestablish its footing, the country’s device makers are aggressively boosting their production goals and aim to expand their output further in the new year. Â
Both in China and elsewhere, memory chip buyers have no choice but to accept higher wafer prices as they race to meet demand from consumers. Moreover, industry sources cited by TrendForce report that inventories across the board are shrinking rapidly, forcing customers to bite the bullet and place even more orders at higher prices. One source is quoted as saying, “Everyone just keeps scrambling for inventory.” Â
However, the longevity of the rising memory prices may be limited. Industry rumors suggest memory manufacturers may be preparing to increase production again in response to downstream demand. While this hasn’t been confirmed by any of the largest memory players, a production uptick does make sense for the first half of 2024.
No one wants a repeat of the sweeping shortages and subsequent round of panic buying seen in the past few years. A stable supply chain is far more beneficial in the long run for all involved. Yet, memory makers also can’t afford to continue selling their chips at incredibly low prices. This is a tricky balance to pull off and it will be interesting to see how the industry handles it in the coming months. Â
Notably, if production increases again, prices will likely slow their rise and stabilize around their current level. In the days ahead, both buyers and manufacturers must carefully monitor memory prices and inventory levels to prevent disruption and ensure profitability.
‍
.jpg)
New Developments Coming in 2024 - December 15, 2023
Partnerships are the name of the game in today’s intertwined and convoluted chip industry. From securing supply chains to advancing manufacturing technology, more chipmakers are teaming up today than ever before. Â
Meta and MediaTek have recently announced a collaboration to develop next-gen chips for AR/VR smart glasses. Meanwhile, Intel has chosen TSMC to produce its Lunar Lake PC chips in a surprising move for its forthcoming mainstream platform. Â
MediaTek, Meta to Collaborate on AR/VR Chips for Next-Gen Smart Glasses
Although artificial intelligence (AI) has dominated headlines for the past few years, many other technologies are also experiencing exciting growth and development. Virtual reality (VR) and augmented reality (AR) have come a long way, primarily thanks to chip advancements that allow them to operate untethered from external computers.
Meta has rapidly cemented itself as a leader in this space and is expected to hold 70% of the overall market share in 2023 and 2024. Both its current Quest VR headsets and more experimental augmented reality glasses are far more refined than models from years past. Thanks to the accelerating adoption of AI, these devices are expected to get much smarter. Â
Now, Meta has announced a collaboration with MediaTek to develop AR and VR semiconductors for its next-gen smart glasses. Notably, Meta has relied on Qualcomm chips until this point to power the last two generations of its smart glasses.
According to TrendForce, the move is likely an effort by Meta to decrease costs and secure its supply chain. Meanwhile, the partnership will help MediaTek challenge Qualcomm in the AR/VR space and expand its footprint.
This fall, Meta introduced the second generation of its smart glasses product—the first came as part of a collaboration with Ray-Ban. The new glasses will feature improved recording and streaming capabilities to further cater to their primary audience of social media users. They will also take advantage of generative AI advancements by integrating Meta’s AI Voice Assistant powered by the Llama2 model. Â
MediaTek’s expertise in building efficient, high-performance SoCs will be essential to this ambition. AR glasses have traditionally been clunky, which has hindered their adoption. A sleeker design that doesn’t sacrifice advanced capabilities could change this. Â
Meta has been working diligently to develop chips in-house, including through a collaboration with Samsung. Its partnership with MediaTek can be seen as a risk management strategy in the short term as it continues to move toward chip independence. As smart glasses currently come with price tags as high as $300 that may deter consumers, the move may also be a way to cut production costs thanks to MediaTek’s competitive pricing. Â
Interestingly, despite the buzz around its AR and VR products, Meta has shipped just 300,000 pairs of its original model. With an anticipated launch of its next-gen smart glasses in 2025, it remains unclear how the market will receive them. Moreover, there are no concrete indications that the AR device market has gained significant traction.
Despite this, MediaTek’s decision to collaborate with Meta extends beyond the latter’s line of devices. By strategically integrating itself into Meta’s chip supply chain, MediaTek can cut into Qualcomm’s dominant market share. In a sense, the move should be viewed as a strategic move for the future rather than a quick way to add revenue now. Should Meta further increase its already-large market share or the general adoption of AR and VR devices increase, MediaTek could benefit greatly. Â
The Taiwanese firm has made a big push into the VR and AR market in recent years and its latest move is a signal that it plans to continue on this path. In 2022, a MediaTek VR chip was used in Sony’s PS VR2 headset. Now, thanks to this partnership with Meta, MediaTek chips could become a foundational part of the AR and VR device market over the coming years. Â
Intel Chooses TSMC’s 3nm Process for Lunar Lake Chip Production
Intel’s upcoming Lunar Lake platform, designed for next-gen laptops releasing in the latter half of next year, is making waves in the PC industry thanks to its innovative design and solid specs. After years of keeping production of its mainstream PC chips in-house, Intel is looking outward for its latest platform. The U.S. chipmaker is reportedly partnering with TSMC for all primary Lunar Lake chip production using the Taiwanese firm’s 3nm process. Â
Notably, neither Intel nor TSMC has directly commented on the partnership after leaks of its internal design details began spreading last month. However, discussions between industry experts on social media and recent reports from TrendForce bring validity to rumors. Â
Lunar Lake features a system-on-chip (SoC) design comprised of a CPU, GPU, and NPU. Then, Intel’s Foveros advanced packaging technology is used to join the SoC with a high-speed I/O chip. A DRAM LPDDR5x module is also integrated on the same substrate. TSMC will reportedly produce the CPU, GPU, and NPU with its 3nm process, while the I/O chips will be made with its 5nm process. Â
Mass production of Lunar Lake silicon is expected to start in the first half of 2024. This timeline matches the resurgence in the PC market most industry analysts project for the back half of next year. After slumping device sales over the past several consecutive quarters, a turnaround is expected thanks to demand for new AI features and a wave of consumers who purchased devices during the pandemic being ready to upgrade. Â
This latest partnership is far from the first time Intel and TSMC have worked together, but the fact that it involves Intel’s mainstream PC chip line is noteworthy. The latter produced Intel’s Atom platform more than ten years ago. However, Intel has only recently started outsourcing chips for its flagship platforms, including the GPU and high-speed I/O chips used in its Meteor Lake platform. Â
According to TrendForce, the decision to move away from in-house production and trust TSMC with the job hints at future collaborations. What this could look like remains to be seen, but TSMC may soon find itself producing Intel’s mainstream laptop platforms more frequently.
‍
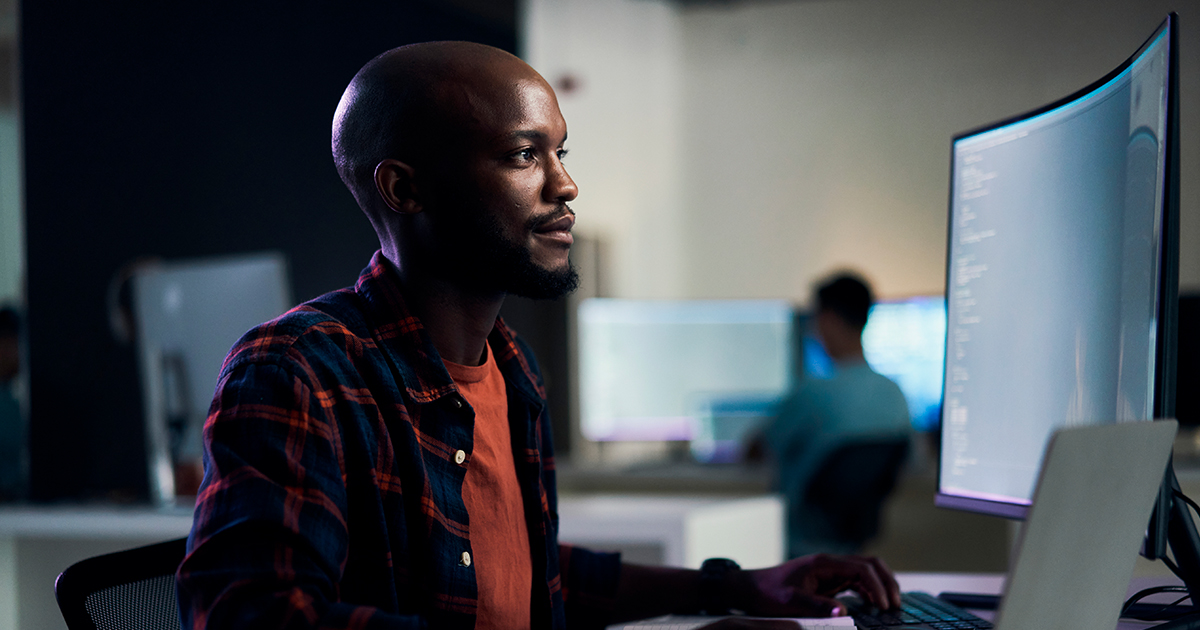
Proactive Sourcing Strategies for All Components - December 1, 2023
The chip industry is positioned for a stretch of significant growth over the coming decade. Technologies like artificial intelligence promise to revolutionize the supply chain and daily life. However, this exciting shake-up means OEMs must carefully strategize the best way to source components—both advanced and legacy node chips—to prevent costly production delays. Â
Meanwhile, companies are racing to improve their AI capabilities. Training new models is a key priority and is being benchmarked with a new test designed for measuring the efficiency of training generative AI. Unsurprisingly, Nvidia leads the way, but both Intel and Google have made big improvements. Â
OEMs Must Prepare to Navigate Supply Chain Shifts Across Node Sizes as Chip Industry Evolves
For original equipment manufacturers (OEMs), the semiconductor supply chain is simultaneously one of the most difficult sectors to source inventory from yet also more accessible than ever. With supply and demand imbalances as well as inventory shortages and gluts raging over the past few years, the industry has been anything but stable. As chipmakers work to expand the production of advanced node chips, legacy nodes are falling out of favor even as demand for them remains steady. Â
This means OEMs must be mindful of sourcing components across node sizes and plan their strategies according to unique trends affecting each one. Over the next decade, technologies like artificial intelligence (AI), 5G, and electric vehicles promise to redefine the chip world. However, the aerospace, defense, and healthcare sectors continue to rely on legacy chips for their essential operations. Â
The latter is likely to cause problems according to many industry analysts. As semiconductor manufacturers shift their production strategies toward more advanced nodes, investment in legacy node fabs has decreased considerably. Certain firms, like GlobalFoundries, have capitalized on this by focusing their efforts on older chips. But the industry is largely moving on.
This means demand for legacy components is poised to outpace supply over the next few years. At a time when many legacy chips are also more difficult to find—often only available through refabrication—OEMs who rely on them are facing an incredibly challenging period for component sourcing. Â
Meanwhile, the majority of chipmakers are being lured in by the high profit margins and demand for cutting-edge chips designed to power AI and EV applications. They continue to invest billions of dollars each year to expand production capacity at existing facilities or build new fabs. The latter takes time, though, often years before a new fab is up and running. OEMs will need to be patient while waiting for shortages to resolve. Â
Moreover, OEMs must act now to adopt forward-looking strategies for sourcing essential components across node sizes. A diverse plan utilizing a more robust network of suppliers is an effective tactic for insulating against the ebbs and flows of the supply chain. This includes working with both local and global suppliers. Many carmakers, particularly those in the EV space, have adopted this tactic by forging relationships directly with chip manufacturers to guarantee inventory. Â
Some experts also recommend OEMs with the capacity to do so purchase extra inventory ahead of potential shortages. While building up a safety stock is expensive, doing so can prevent costly production delays down the line. Of course, on a larger scale, this trend can also further disrupt the supply chain as inventory gluts push down prices—as is currently being seen in the memory chip market. Â
Regardless of the approach OEMs take, the key is to remain agile. Being ready to adapt to changes in the market at a moment’s notice and predict them before they happen is essential in today’s fast-paced market. Utilizing advanced analytics tools and data from every point on the supply chain creates a bigger picture and allows for more accurate decision making. Â
Over the next several years, the chip industry is prepared to through another period of rapid growth and change thanks to AI and electric cars. As demand for different nodes changes with it, OEMs must be prepared to evaluate and adjust their sourcing strategies at multiple levels or risk falling behind. Meanwhile, OEMs relying on legacy components must be prepared to face a shortage and adopt clever solutions to secure enough inventory.
Nvidia Continues to Dominate Generative AI Training, But Intel and Google are Closing In
Training generative artificial intelligence (AI) systems is no easy task. Even the world's most advanced supercomputers take days to complete the process—a timeline few other projects can claim. Less advanced systems require months to do the same work, putting into perspective the vast amount of computing power needed to train large language models (LLMs) like GPT-3. Â
To benchmark progress in LLM training efficiency over time, MLPerf has designed a series of tests. Since launching five years ago, the performance of AI training in these tests has improved by a factor of 49 and is now faster than ever. MLPerf added a new test for GPT-3 earlier this year and 19 companies and organizations submitted results. Nvidia, Intel, and Google were the most noteworthy among them. Â
Each of the three took the challenge quite seriously and devoted massive systems to running the test. Nvidia’s Eos supercomputer was the largest and blew competitors away as expected. The Eos system is comprised of 10,752 H100 GPUs—the leading AI accelerator on the market today by practically every metric. Up against MLPerf’s GPT-3 training benchmark, it completed the job in just four minutes. Â
Eos features three times as many H100 GPUs as Nvidia’s previous system, which allowed it to achieve a 2.8-fold performance improvement. The company’s director of AI benchmarking and cloud computing, Dave Salvatore, said, “Some of these speeds and feeds are mind-blowing. This is an incredibly capable machine.” Â
To be clear, the MLPerf test is a simulation that only requires the training to be completed up to a key checkpoint. The computer reaching it means the rest of the training could have been completed with satisfactory accuracy without the need to actually do it. This makes the test more accessible for smaller companies who don’t have access to a 10,000+ GPU supercomputer while also saving both computing resources and time. Â
Given this design, Nvidia Eos’ time of four minutes means it would take the system about eight days to fully train the model. A smaller system built with 512 Nvidia H100 GPUs, a much more realistic amount for most supercomputers, would take about four months to do the same task. Â
While it should come as no surprise that Nvidia is dominating the AI space given its prowess in the AI chip sector, Intel and Google are making strides to close the gap. Both firms have made big improvements in their generative AI training capabilities in recent years and posted respectable results in the MLPerf test. Â
Intel’s Guadi 2 accelerator chip featured its 8-bit floating-point (FP8) capabilities for the test, unlocking improved efficiency over preceding versions. Enabling lower precision numbers has led to massive improvements in AI training speed over the past decade. It’s no different for Intel, which has seen a 103% reduction in time-to-train. While this is still significantly slower than Nvidia’s system, it’s about three times faster than Google’s TPUv5e. Â
However, Intel argues that the Gaudi 2 accelerator’s lower price point keeps it competitive with the H100. From a price-to-performance standpoint, this is valid. The next-generation Gaudi 3 chip is expected to enter volume production in 2024. Given that it uses the same manufacturing process as Nvidia’s H100, hopes are high for its performance. Â
Over the coming years, the importance of generative AI training will only grow. Advancements like those being made by Nvidia, Intel, and Google are expected to pave the way for a new age of computing. Thanks to benchmarks like MLPerf’s training tests, we can watch the results come through in real-time.
‍
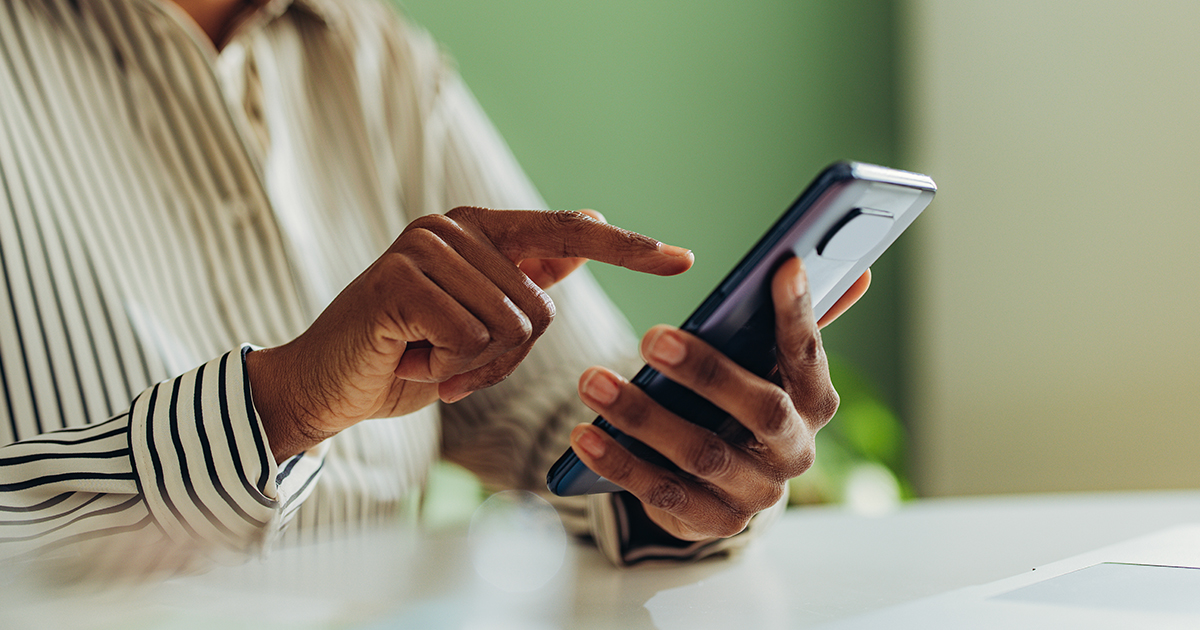
‍AI Helping Pull Memory and Mobile Out of the Depths - Nov 17, 2023
Experts have been quick to tout the revolutionary potential of artificial intelligence (AI). So far, the technology is living up to the hype. Industries across the spectrum are prioritizing AI in their forward-looking plans and chip suppliers are racing to keep up with demand being sparked by the rise of new AI products. Â
For the oft-beleaguered memory chip industry, this growth is a welcome change—and is driving price increases in the fourth quarter. Meanwhile, smartphone makers are working to implement generative AI into their latest devices as industry leaders claim the tech could be as influential as smartphones themselves. Â
DRAM, NAND Prices to Rise in Q4 with Continued Growth in Q1 Next Year
The memory chip market has gone through plenty of turmoil over the last few years. For the past several months, experts have been pointing to signs of recovery even as the industry seemed to bottom out. Now, thanks to numerous market influences, the numbers are starting to echo this sentiment as the memory sector heads for a turnaround in the new year. Â
According to a recent TrendForce report, Q4 memory chip prices are expected to rise by double digits. Mobile DRAM, interestingly, is leading the way with a projected increase of 13-18%. Meanwhile, NAND Flash isn’t far behind with prices for eMMC and UFS components expected to jump by 10-15% in the quarter. Â
Typically, mobile DRAM takes a backseat to traditional DRAM chips, but several factors are buoying its price in the fourth quarter. One of the largest is Micron’s decision to hike prices by more than 20% for many of its leading memory products. Samsung’s recent move to slash production in response to an industry-wide supply glut is also driving memory chip prices upward. Â
Meanwhile, the fall/winter peak season for new mobile devices tends to foster a favorable end of the year for memory chip makers. While this is a factor in the Q4 price rise, it isn’t the only one. Growth in the Chinese smartphone market—sparked by Huawei’s new Mate 60 series—is causing device makers to increase their production targets as consumer demand rises. Notably, the Mate 60 Pro features a 7nm 5G chip, making it the first such Chinese-made device to hit the market since global trade restrictions aimed to cut the country off from advanced chips and chipmaking equipment were implemented. Â
The wider chip industry is also prepared for a positive fourth quarter on the back of increasing electronics sales and IC sales. Notably, this is expected to drive year-over-year growth for key chip markets in Q4 following a stretch of declines over the previous five quarters. Â
Looking ahead, experts don’t expect the memory chip market’s resurgence in the fourth quarter to fizzle out in the new year. The first frame of 2024 promises to be another strong period of growth. Though TrendForce reports, expectations should be tempered since external factors like the Lunar New Year and off-season production lulls will likely slow the rise of prices. Â
Even so, experts believe demand will continue into the new year and that suppliers will maintain their conservative production strategies. Both will influence prices to stay high into Q1 2024 as the memory market continues its impressive recovery.
Xiaomi First to Smartphone Generative AI Features with Qualcomm’s Latest Snapdragon Chip
There was a time when foldable displays and 5G were expected to be turning points for smartphones. In a sense they were, but those technologies are already on the edge of becoming outdated thanks to the arrival of artificial intelligence (AI).
Qualcomm’s Snapdragon 8 Gen 3 mobile chipset launched in late October boasting on-device generative AI, which dramatically speeds up processing-intensive activities usually done on the cloud. Xiaomi simultaneously announced new flagship phones using the edge AI Qualcomm mobile platform.
Generative AI has boomed this year thanks in large part to the rise of ChatGPT. Its uncanny ability to create shockingly passable content in response to simple user prompts sets it apart from other forms of AI that require an expert-level understanding to use productively. Tech luminaries like Bill Gates and Sundar Pichai have said they think generative AI on smartphones could be as big as the dawn of the internet and smartphone technology itself.
Xiaomi founder and president Lu Weibing was the sole smartphone manufacturer to speak at the 2023 Qualcomm Snapdragon Technology Summit, where he showcased the Xiaomi 14 series. Â
"Xiaomi and Qualcomm have a long-term partnership, and Xiaomi 14 demonstrates our deep collaboration with Qualcomm. This is one of the first times a new platform and device launch together," Lu said.
The Xiaomi 14 and 14 Pro, presently exclusive to China pending a global launch, have AI capabilities ranging from those considered fairly basic, like summarizing webpages and generating videoconferencing transcripts, to ambitious features such as inserting the user into worldwide location and event scenes.
Qualcomm claims in its press release that Snapdragon 8 Gen 3 ushers in a new era of generative AI, “making the impossible possible.” The platform’s large language models (LLM) can run up to 20 tokens/sec—one of the fastest benchmarks in the smartphone industry—and generate images in a fraction of a second.
"AI is the future of the smartphone experience," said Alex Katouzian, senior vice president and general manager of Qualcomm's mobile, computing, and XR division.
Xiaomi has been working on AI since 2016 and maintains a dedicated AI team of over 3,000 people. Between that team and more than 110 million active users worldwide, Xiaomi’s digital assistant Xiao AI, which got upgraded with generative AI capabilities in August 2023, has the potential to continue evolving rapidly.
The digital assistant recognizes songs and objects, prevents harassing calls, suggests travel routes, and provides medication reminders. It can also control household appliances when integrated into Xiaomi’s line of smart home devices.
Xiaomi is the world's leading consumer IoT platform company and quietly the world’s third-largest smartphone manufacturer. The company connects 654 million smart devices and commands an impressive 14% global smartphone market share. Of course, its smartphone rivals in China have also been busy in the AI arena. Huawei, Oppo, and Vivo recently announced major upgrade plans for their own digital assistants.
The recently launched Google Pixel 8 also focused on AI with the company boasting its algorithms’ ability to pick out the best facial expressions in batches of group photos and easily paste them into a different image. That allows, for example, for a photograph to feature eight happy, smiling people when only four were actually smiling when the shutter snapped. Magic Compose, another feature that Google announced at its May 2023 developer conference, uses generative AI to suggest responses to text messages or rewrite responses in a different tone. Â
According to a recent report from Bloomberg, Apple is said to be developing a number of new AI features for the iPhone and other iOS products. Scheduled for release in 2024, iOS 18 is expected to include AI upgrades to Siri, Apple’s messaging app, Apple Music, Pages, Keynote, and more.
"There may be a killer use case that doesn't exist yet," said Luke Pearce, a senior analyst for CCS Insight, a tech research and advisory firm. "But that will come around the corner, surprise us all, and become completely indispensable.”
In the meantime, generative AI promises to be the smartphone industry’s next major milestone. As the technology continues to evolve, expect device makers to find more ways to incorporate it while taking advantage of the latest edge AI semiconductors.
‍

New Shortage Coming for AI? - Nov 3, 2023
Artificial intelligence (AI) is arguably today’s most exciting technology. However, as with any new tech, adoption and development aren’t easy. Chipmakers and buyers alike are currently struggling with a shortage of AI components due to bottlenecks in advanced chip production lines at TSMC and SK Hynix. Those are expected to ease in 2024 thanks to aggressive capacity upgrades as AI represents a big opportunity for chip companies.
Meanwhile, Nvidia dominates the AI market thanks to its combined offerings of both hardware and software. Companies like AMD are attempting to challenge Nvidia’s power by exploring new software solutions—and acquiring startups who have done the same—to pair with their existing hardware offerings. Â
Production Bottlenecks Affecting the Chip Industry Amid AI Boom
The number of artificial intelligence (AI) applications has skyrocketed as generative AI takes the world by storm. Businesses of all shapes and sizes are exploring ways to integrate the technology into their operations—and use it to boost their bottom lines. However, the advanced chips needed to power large language models (LLMs) and AI algorithms are complex and difficult to produce.
Take Nvidia’s flagship H100 AI accelerator, for instance. Though orders for the chip are piling up, production is limited by the fact that TMSC is the only firm manufacturing the H100. As a result, availability of the GPU is limited by TSMC’s tight production capacity, particularly in CoWoS packaging. While the Taiwanese chipmaker expects to start filling orders in the first half of 2024, this bottleneck represents a larger issue for the AI industry.
As demand for AI products soars, chipmakers are scrambling to keep up. High-powered GPUs aren’t the only components experiencing bottleneck issues either. The smaller components inside them are also difficult to source given their novelty and scarcity. Â
HBM3 chips, the fastest memory components needed to support AI’s intense computations in the H100, are currently supplied exclusively by SK Hynix. The latter is racing to increase its capacity, but doing so takes time. Meanwhile, Samsung is hoping to secure memory chip orders from Nvidia by next year as it rolls out its own HBM3 offerings. Â
All said, data from DigiTimes points to a massive disparity between supply and demand in the AI server market. Analyst Jim Hsiao estimates the current gap is as wide as 35%. Even so, more than 172,000 high-end AI servers are expected to ship this year. Â
This move will be supported by chipmakers who have made (and continue making) significant increases in their production capacity. By mid-2024, TSMC’s CoWoS capacity is expected to increase to around 30,000 wafers per month—up from the 20,000 wafer capacity it projected for the new year this summer. Â
Demand for AI servers is led by massive buying initiatives from tech firms as they seek to revamp their computing operations with AI. Indeed, 80% of AI server shipments are sent to just five buyers—Microsoft, Google, Meta, Amazon (AWS), and Supermicro. Of these, Microsoft leads the way with a staggering 41.6% of the total market share for high-end AI servers. Â
Analysts believe this head start will make unseating Microsoft from its position of AI leadership very difficult in the coming years. A novel breakthrough in new technology or significant investment will be needed for the likes of Google and Meta to catch up given their respective 13.5% and 10.3% market shares. Â
Notably, the growing demand for AI servers is also causing a drop in shipments of traditional high-end servers. Top tech firms are moving away from these general-purpose machines as their budgets are pulled in multiple directions. Moreover, companies are choosing to buy directly from ODM suppliers rather than server brand manufacturers like Dell and HP. Experts predict ODM-direct purchases will account for 81% of total server shipments this year. This change has forced suppliers to adapt quickly to their business model being ripped out from under them. Â
Competition in the AI space is expected to heat up in 2024 and beyond thanks to capacity increases and profitable applications for AI continuing to be discovered. As the necessary hardware becomes more readily available, buyers will have more options and flexibility in their orders, leading to more competition among manufacturers. Though AI demand is likely to even out over time, this sector represents a massive opportunity for chipmakers over the coming decade. Expanding advanced chip production capacity is step one toward reliably fueling the AI transformation. Â
AMD Acquires Nod.AI to Bolster its AI Software Portfolio and Compete with Nvidia
In the race for dominance in the artificial intelligence (AI) sector, all roads run through Nvidia. The GPU maker saw its revenue spike by over 100% this year on the back of significant demand for AI products and its decade-long foresight to position itself as a market leader. While Nvidia’s hardware is certainly impressive, its fully-fledged ecosystem of AI software and developer support is what truly gives the firm such a massive advantage. Â
In an effort to catch up, AMD has announced its acquisition of Nod.AI. The startup is known for creating open-source AI software that it sells to data center operators and tech firms. Nod.AI’s technology helps companies deploy AI applications that are tuned for AMD’s hardware more easily. While details of the acquisition weren’t disclosed, Nod.AI has raised nearly $37 million to date. Â
In a statement, an AMD spokesperson said, “Nod.AI’s team of industry experts is known for their substantial contributions to open-source AI software and deep expertise in AI model optimizations.” Â
Nod.AI was founded in 2013 by Anush Elangovan, a former Google and Cisco engineer. He was joined by several noteworthy names from the tech industry, including Kitty Hawk’s Harsh Menon. The startup launched as an AI hardware company focusing on gesture recognition and hand-tracking wearables for gaming. However, it later pivoted to focus on AI deployment software, putting it closely in line with AMD’s needs. Â
The startup will be absorbed into a newly formed AI group at AMD which was created earlier this year. Currently, the group consists of more than 1,500 engineers and focuses primarily on software. AMD already has plans to expand this team with an additional 300 hires in 2023 and more in the coming years. It’s unclear if these figures include the employees coming in from Nod.AI or if AMD plans to hire even more staff in addition to them. Â
Nod.AI’s technology primarily focuses on reinforcement learning. This approach utilizes trial and error to help train and refine AI systems. For AMD, the acquisition is another tool in its belt of software offerings to tempt prospective buyers with. Reinforcement learning tools help customers deploy new AI solutions and improve them over time. With software designed to work well with AMD’s hardware, the process becomes more intuitive and leads to faster launch times. Â
While AMD is a long way from being able to compete with Nvidia’s software platform and developer support directly, it is taking steps in the right direction. The company is utilizing both internal investment and external acquisitions to do so, according to its president Victor Peng. Nod.AI is its second major acquisition in the AI software space in the past few months. Though AMD has no current plans for further moves, Peng noted that the firm is “always looking.” Â
Over the coming years, Nvidia’s lead in the AI space will be challenged. For AMD and others, combined innovation in both hardware and software will be essential to stealing market share from the current leader. AMD’s latest acquisition will help bolster its software portfolio in the short term with immediate effect while it continues to scale its long-term plans.
‍
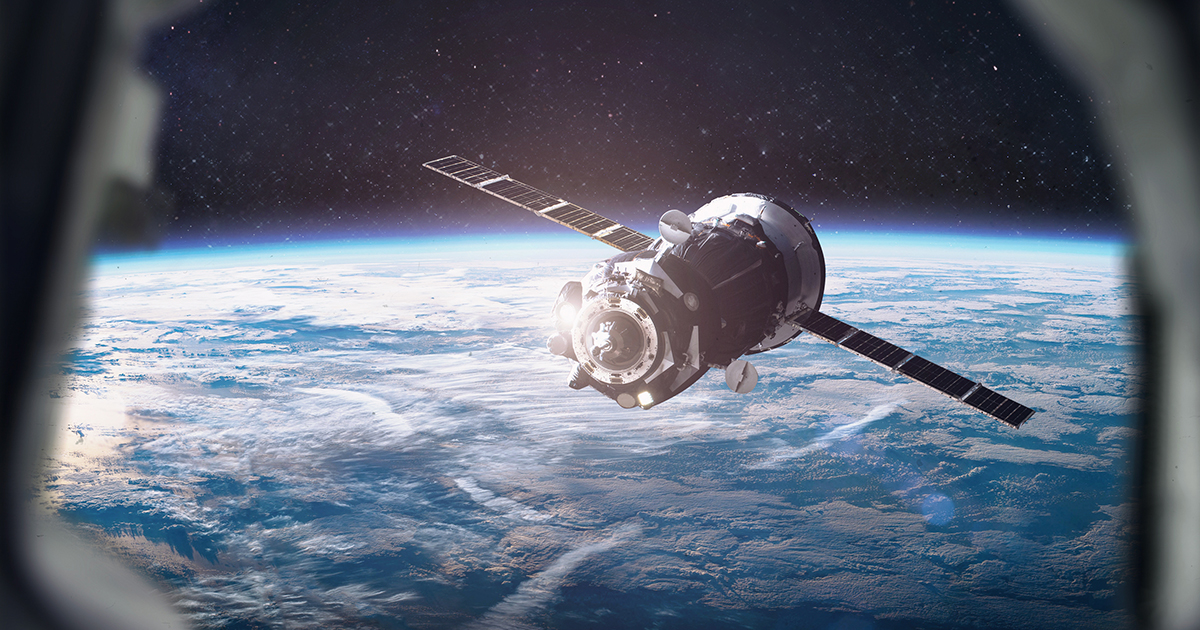
Leaders Pledge AI Protection as Satellites Make Chips in Space - October 20, 2023
The chip industry is already worth $500 billion and that figure is expected to double by the end of the decade. With so much on the line chipmakers are tech companies are doing everything they can to innovate and evolve. Â
For some, this means pursuing the mind-boggling power of artificial intelligence. With many still wary about the technology, though, government officials and tech leaders are teaming up to safely advance the AI field and earn trust. Others are looking elsewhere for innovation—including the stars. Could manufacturing semiconductor materials in space be the key to more efficient chips in the years to come? One U.K. startup thinks the answer is yes. Â
Tech Leaders Commit to Safely Advancing AI Through Collaboration and Transparency
It’s no secret artificial intelligence (AI) is reshaping the way the world interacts with technology. Thanks to the introduction of ChatGPT, machine learning and generative AI have gone mainstream with everyone from leading tech firms to average end users experimenting with its possibilities. But some are less enthusiastic about the potential applications for AI and remain wary of its risks. Â
As the ubiquity of AI extends to practically every industry, tech leaders and government officials are coming together to pledge their commitment to AI safety. Spearheaded by the Biden Administration, the voluntary call-to-action has been answered by 15 influential tech firms in recent months. Â
Earlier this year, the program was initially backed by seven AI companies. This included Google, Microsoft, OpenAI, Meta, Amazon, Anthropic, and Inflection. Now, eight more firms have pledged their intention to aid in the safe development of AI, including Adobe, IBM, Nvidia, Palantir, Salesforce, Cohere, Stability, and Scale AI. Â
The latter said in a recent blog post, “The reality is that progress in frontier model capabilities must happen alongside progress in model evaluation and safety. This is not only the right thing to do, but practical.” Â
“America’s continued technological leadership hinges on our ability to build and embrace the most cutting-edge AI across all sectors of our economy and government,” Scale added.
Indeed, companies around the world are exploring new ways to integrate artificial intelligence into every aspect of their operations. From logistics to customer service and research to development, AI promises to revamp the way work is done. Â
Even so, the safety concerns of AI loom large over any potential benefits. Much of this fear stems from not understanding how the models themselves work as they grow larger and more complex. Others fear it will soon be impossible to differentiate AI-generated content from human-generated work. Meanwhile, the cybersecurity risks associated with AI are numerous while solutions remain largely unexplored. Â
The Biden Administration’s plan to address these problems is threefold. Step one puts an emphasis on building AI systems that put security first. This includes investment in cybersecurity research and safeguards to prevent unwanted access to proprietary models. AI firms involved in the pledge have also committed to rigorous internal and external testing to ensure their products are safe before introducing them to the public. Given the rapid advancement of AI and industry-wide push to bring new applications to market first, this is an important guardrail. Â
Finally, the companies involved have made a commitment to earning the public’s trust. They aim to accomplish this in many ways, but ensuring users know when content is AI-generated is paramount. Other transparency guidelines include disclosing the capabilities of AI systems and leading research into how AI systems can affect society. Â
In a statement, the White House said, “These commitments represent an important bridge to government action, and are just one part of the Biden-Harris Administration’s comprehensive approach to seizing the promise and managing the risks of AI.” Â
The statement also mentions an executive order and bipartisan legislation currently being developed. Ultimately, though, no one company or government will dictate the future of AI. An ongoing collaboration is needed to ensure the technology is developed safely over the coming years. Â
Getting the most influential tech firms on board early is a big step in the right direction. Closely monitoring their actions to ensure they consistently align with this commitment is crucial as more innovations are made and AI continues to evolve. Â
U.K. Startup Eyes Satellite-Based Chip Manufacturing in Space, Promises Greater Efficiency
Producing high-quality semiconductors requires a precise manufacturing environment that costs billions of dollars to create. While the microgravity and vacuum of outer space are hostile to human life, they are perfect for making semiconductors. Some companies, including U.K.-based startup Space Forge, are exploring the possibility of manufacturing chips in orbit. Â
The startup’s ForgeStar-1 satellite is on its way to the U.S. and will be launched either late this year or early next year. This comes after its first attempt at a launch went awry when the Virgin Orbit rocket it was strapped to failed in January. Â
The satellite is roughly the size of a microwave but contains a powerful automated chemistry lab. Researchers on Earth will control the devices inside to mix chemical compounds and experiment with novel semiconductor alloys. They’ll be able to monitor how the substances behave in microgravity and the vacuum of space compared to their responses on Earth. Â
In a statement to Space.com, Space Forge CEO Josh Western said, “Producing compound semiconductors is a very intense and very slow process, they are literally grown by atoms, and so gravity has a profound effect… In space you’re able to overcome that barrier, because there is an absence of gravity.” Â
Of course, microgravity isn’t the only advantage of making chips in space. The process also benefits from the perfect vacuum—something chipmakers rely on expensive machinery to replicate on Earth to protect materials from contamination. Manufacturing chip materials in space negates the need for manmade vacuum equipment and ensures contaminants are non-existent. Â
Space Forge estimates that the favorable conditions of outer space make it possible to produce chips that are 10 to 100 times more efficient than those made on Earth. That’s a notable improvement that could have radical implications for the $500 billion semiconductor industry—especially considering that its size is expected to double by 2030.
Of course, making semiconductors in space isn’t as simple as it sounds. Materials produced in orbit would need to be safely returned to Earth for further processing and packaging. Protecting such sensitive materials on a rough and fiery trip back into the atmosphere is a major challenge. Â
Space Forge’s first satellite won’t even attempt this feat. Instead, it will beam experiment data back to Earth digitally for researchers to analyze. The startup does plan to return its satellites eventually, but Western says this isn’t in the forecast for another two to three years. Â
Although the prospect of making chips in space remains futuristic, this is an exciting development to monitor. Manufacturing semiconductors in space’s favorable environment could yield far more efficient chips in the years to come. As chipmakers seek new ways to produce the most advanced silicon and cash in on the growing industry’s demand, no approach is too outlandish to consider.
‍
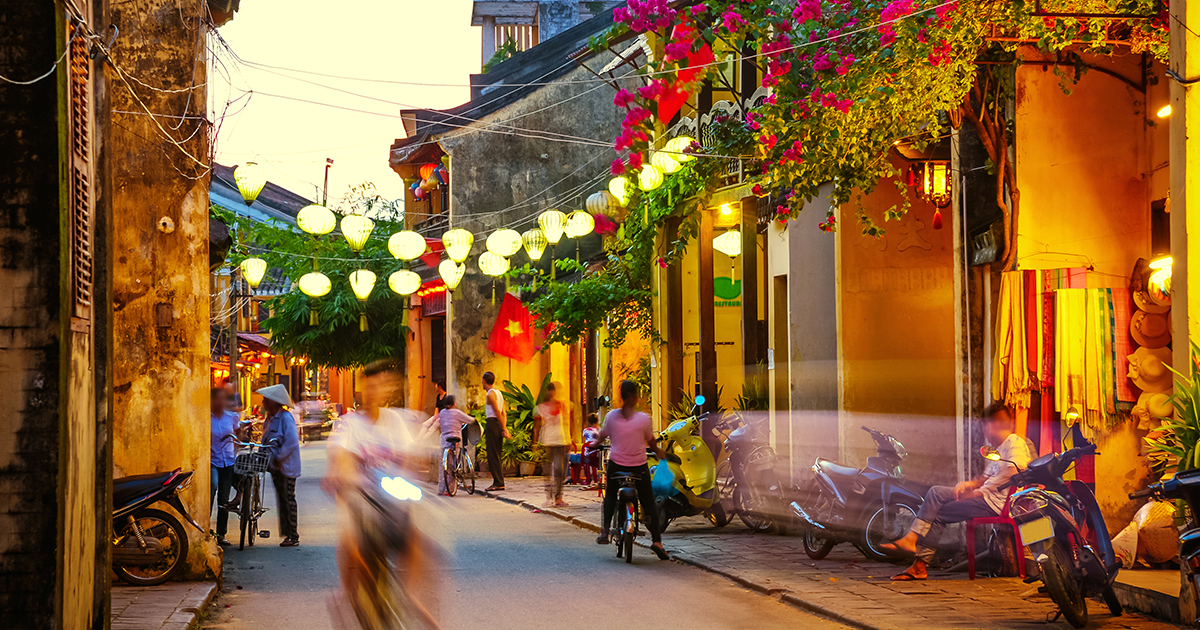
Moving Forward with Semiconductor Development – October 3, 2023
As tensions with China persist, chipmakers are looking for new ways to diversify their supply chains. This has put developing Asian nations in the spotlight. Perhaps none have shined as brightly as Vietnam, which the U.S. views as a key strategic partner. A major tech summit last month saw several billion-dollar business partnerships with U.S. chip firms inked. Â
Meanwhile, U.S. domestic chip ambitions remain strong. Private companies and public institutes alike are working to advance the speed of American chip innovation while also bolstering a workforce facing massive shortages. A new $46 billion investment from the National Science Foundation aims to address both of these goals as the U.S. continues expanding its stake in the chip sector. Â
Vietnam, U.S. Strengthen Ties Amid New Semiconductor Deals
Political and economic relations between the U.S. and Vietnam have been icy over the past few decades. However, this sentiment is changing thanks to hard work from government officials on both sides. The two countries have now agreed to billions of dollars in business partnerships following a major tech summit and diplomatic visit by U.S. President Joe Biden. Â
As countries and companies alike seek to diversify their supply chains away from China, developing nations in Asia have taken center stage. Thanks to cheap labor and regional accessibility to other chip operations, these countries offer the most convenient path to a more stable supply chain. Vietnam, which now sees the U.S. as its largest export market, has seized the opportunity to expand its role in the chip sector. Â
In a press conference, President Biden said, “We’re deepening our cooperation on critical and emerging technologies, particularly around building a more resilient semiconductor supply chain.”
“We’re expanding our economic partnership, spurring even greater trade and investment between our nations,” he added. Â
A number of key government officials joined executives from top tech and chip firms, including Google, Intel, Amkor, Marvell, and Boeing, at the Vietnam-U.S. Innovation and Investment Summit last month. The roundtable consisted of discussions on how to deepen partnerships and spark new investments.
Those talks are already paying dividends as several new business deals were announced. Leading the way was a $7.8 billion pledge from Vietnam Airlines to purchase 50 new 737 Max jets from Boeing. Â
In the chip sector, two new semiconductor design facilities are being built in Ho Chi Minh City. One will belong to Synopsys and the other to Marvell—both U.S.-based firms. At a broader level, the partnership seeks to “support resilient semiconductor supply chains for U.S. industry, consumers, and workers.”
These deals come shortly after Amkor announced a $1.6 billion chip fab near Vietnam’s capital of Hanoi. The company expects its new facility, which will primarily be used for assembly and packaging, to open sometime this month.
Despite this positive momentum, a dark cloud still hangs over the partnership. Vietnam’s chip workforce remains concerningly small. Currently, just 5,000 to 6,000 of the country’s roughly 100 million citizens are trained hardware engineers. Demand forecasts for the next five years project Vietnam will need 20,000 hardware engineers to fill an influx of new chip jobs. That number will more than double to 50,000 in ten years. Â
Without a robust pipeline of new chip talent, even the loftiest partnerships and investments could be in jeopardy. This reflects a similar chip worker shortage in the U.S., where 67,000 positions for technicians and engineers are expected to go unfilled by the end of the decade. Â
Even so, the increase in collaboration between Vietnam and the U.S. is a positive sign. Chipmakers are working to quickly decouple their supply chains from China as economic tensions with the country and its international trade partners sour. Hubs like Vietnam, India, and Malaysia have become central to these efforts. With each bit of political momentum, relocating chip production becomes easier. Â
The U.S.-Vietnam partnership is an important one to monitor in the coming months. Both Washington and Hanoi believe their new strategic partnership will usher in even more investment deals than those already revealed. Whether or not the latest wave of diplomacy is enough to entice investors and other chipmakers remains to be seen. Â
National Science Foundation Commits $45.6M to Support US Chip Industry
In a new wave of support arriving as a result of the CHIPS Act, the U.S. National Science Foundation (NSF) has pledged $45.6 million to support the domestic semiconductor industry. The public-private partnership brings in several top firms, including IBM, Ericsson, Intel, and Samsung. Notably, the support comes through the NSF’s Future of Semiconductors (FuSe) program. Â
NSF Director Sethuraman Panchanathan said in a statement following the investment, “By supporting novel, transdisciplinary research, we will enable breakthroughs in semiconductors and microelectronics and address the national need for a reliable, secure supply of innovative semiconductor technologies, systems, and professionals.” Â
The program funds are divided across 24 unique research and education projects with more than 60 awards going to 47 academic institutions. The grants are funded primarily by the NSF using dollars from the CHIPS Act with additional contributions coming from the partner companies. Each firm has pledged to provide annual support for the program through the NSF. Â
NSF leaders hope the investment will serve as a catalyst for chip breakthroughs. The FuSe program emphasizes a “co-design” approach to chip innovation, which considers the “performance, manufacturability, recyclability, and environmental sustainability” of materials and production methods.
Perhaps more important to U.S. semiconductor ambitions, though, is developing a robust chip workforce. Currently, the nation is lacking in this area with 67,000 chip jobs expected to be vacant by 2030. The NSF’s latest investment places a heavy emphasis on developing chip talent in America. As the industry works to shore up workforce gaps, collaboration is more essential than ever. Â
Panchanathan says the NSF’s $46 million investment will “help train the next generation of talent necessary to fill key openings in the semiconductor industry and grow our economy from the middle out and bottom up.” Â
Leveraging the expertise and support of industry partners is a key component of the FuSe program. Dr. Richard Uhlig, senior fellow and director of Intel Labs, said in a joint press release, “Implementing these types of programs across the country is an incredibly powerful way to diversify the future workforce and fill the existing skills gap.” Â
Meanwhile, President of Samsung Semiconductor in the U.S., Jinman Han, said, “Helping drive American innovation and generating job opportunities are critical to the semiconductor industry… As we grow our manufacturing presence here [in the U.S.], we look for partners like NSF that can help address the challenges at hand and drive progress in innovation while cultivating the semiconductor talent pipeline.” Â
Demand for semiconductors is growing around the world. As chipmakers seek to move their operations out of China amid tense economic conditions, the U.S. domestic industry is vying to reclaim its status as a global powerhouse. The CHIPS Act, passed in 2022, has sparked a new period of growth and innovation as demonstrated by initiatives like this one. Â
As the latest wave of NSF-funded projects roll out over the coming months, it will be exciting to see what sort of innovation they yield. Likewise, these essential programs will help inspire the next generation of chip talent in America.
‍

Corporate Demand for AI is Driving Chip Market - September 19, 2023
The chip market has endured a tumultuous few years in the wake of the COVID-19 pandemic. Luckily, several factors within the tech industry are paving the way for a strong recovery and pattern of growth. From IoT devices to automakers eyeing electric vehicles, products across every sector need more chips. As the AI craze reaches full swing, chipmakers are turning record profits and preparing to use this as an opportunity for bolstered growth in the coming years. Â
AI, Increasing Tech Adoption Drive Chip Demand, Grow Market
The semiconductor industry appears well-positioned for a period of growth according to a report from the IMARC group which looks out as far as 2028. IMARC predicts the chip industry will reach $941.2 billion globally by 2028 and experience a growth rate (CAGR) of 7.5% from now until then. Â
So, what’s driving this steady incline for the industry? As the global economy leans more heavily on tech-centered products, the need for semiconductors rises. Given a push for more technology in offerings across sectors, chips are increasingly in demand—even in places they haven’t been traditionally. Â
The growing Internet of Things (IoT) is one major driver of growth. More and more of today’s devices are connected to the internet, enabling efficient communication and seamless collaboration. Of course, these devices require several components to stay connected, going beyond those required for their core function. Analysis firm Mordor Intelligence projects the IoT chip market to reach $37.9 billion by 2028, expanding significantly from its current $17.9 billion size. Â
Meanwhile, the automotive industry is also hungry for chips thanks primarily to the rise of electric vehicles (EVs). These cars rely on more components to power their complex software and hardware. Semiconductors are essential for key EV features like battery control, power management, and charging. Even non-electric vehicles are being built with more chips today than ever before. Consumers demand flashy, attractive features, which increases the number of chips needed to support them. As some companies lean into self-driving technology and advanced driver assistance programs, more advanced chips are required. Â
Indeed, the automotive industry has been one of the biggest chip buyers in recent years. Experts predict this trend will only increase as more carmakers and governments emphasize EVs, citing environmental concerns. Analysts project the auto sector will be the third largest buyer of semiconductors by 2030, accounting for $147 billion in annual revenue. Â
Artificial intelligence (AI) is perhaps the most influential technology in the world right now. Surging demand has chipmakers fighting to keep up as companies race to invest in AI. The rise of ChatGPT sparked interest in generative AI, catching the eye of major tech players like Microsoft and Google. Meanwhile, other machine learning applications are being explored across every industry, driving demand for high-power data center chips. Benzinga projects the
AI chip market will grow to $92.5 billion by 2028, a staggering increase from the $13.1 billion it was valued at in 2022. Â
Nivida has a massive head start on the market and currently is responsible for making the overwhelming majority of AI chips thanks to its prowess in the GPU market. Memory chipmakers are also benefiting as AI applications require high-speed DRAM units. The explosive growth of AI is driving demand for HBM3 memory chips and their successors. Â
Finally, an increased desire for high performance across devices has put chipmakers in a favorable position. Emerging technologies such as 5G and edge AI computing require advanced silicon and additional components to enable connectivity.
As the world continues to embrace technology in every facet of daily life, the chip industry must be ready. Addressing critical workforce shortages and ensuring manufacturing capacity is sufficient are key areas to watch in the coming years. Moreover, creating a more diverse supply chain that is more resilient in the face of economic disruption is essential. By working to solve these challenges, the chip industry can put itself in a place to succeed throughout the remainder of the decade. Â
Corporate Demand for ChatGPT is an Excellent Opportunity for Chipmakers
ChatGPT, the flagship offering of OpenAI, has revolutionized the way the world thinks about artificial intelligence (AI). The technology has started evolving at a rapid pace—and the industry powering it isn’t far behind. As tech giants gear up for an AI arms race over the next decade, chipmakers are racing to meet demand. Â
Now, OpenAI is working to monetize its AI golden child. The Microsoft-backed startup reportedly brings in $80 million each month. But it isn’t content to stop there. OpenAI recently introduced a new ChatGPT business offering, which gives corporate clients more privacy safeguards and additional features. The so-called ChatGPT Enterprise comes via a premium subscription which the company has not yet provided pricing details for. Â
OpenAI reportedly worked with more than 20 companies to test the product and find the most marketable solutions. Zapier, Canva, and Estee Lauder were all involved and remain early customers of the product. However, OpenAI claims that over 80% of Fortune 500 companies have also used its software since its launch late last year. Â
For the chip industry, AI represents a turning point. The technology is already being used to make chip manufacturing more efficient. Startups have tasked AI-powered computer vision programs with spotting defects in wafers during production. This method is both faster and more accurate than manually reviewing each wafer, resulting in greater revenue and production for chipmakers. Â
Elsewhere, researchers are beginning to explore how applying machine learning principles to the intricacies of chip design could one day result in more efficient, more powerful semiconductors than humans can create. Samsung is a pioneer in this area. The South Korean firm is already employing generative AI in hopes of competing with TSMC by increasing wafer yields. Â
Despite these promising applications, data from McKinsey shows just 30% of companies who currently use AI and machine learning see value from it. But this is expected to change quickly as more businesses experiment with AI and learn how to utilize it most effectively. The same report suggests the use of AI could generate $85 to $95 billion in the long term. Â
For chipmakers, using AI in-house isn’t the only factor to consider. As the world’s largest firms scramble to gain an advantage in the AI gold rush, their need for high-performance chips is dire. Without the right hardware, it’s impossible to train AI models and use them to generate and analyze profitable data. Firms who are able to provide the needed silicon will benefit tremendously. Â
As ChatGPT is so often an indicator of the wider AI market’s direction, don’t be surprised to see a big push to include AI in the office in the coming months. Startups of all sizes will introduce their offerings to businesses seeking to improve their efficiency and processes. Each day, more “real-world” uses for AI will crop up as startups who have hungrily devoured capital seek to start turning a profit. For chipmakers, the winners of the AI race aren’t what matters. Rather, the success or failure of AI as a concept and as a useful technology will dictate much of what the future looks like for chips. With some luck, it will be a key growth driver for the industry over the next ten years.
‍
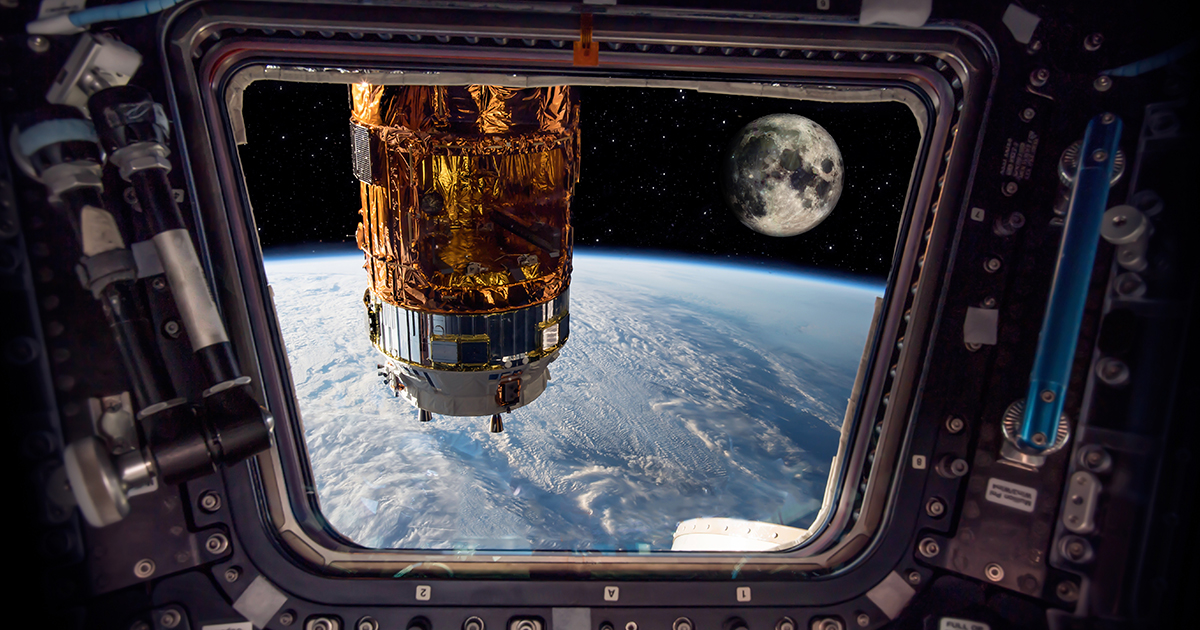
Semiconductors in Space! - September 5, 2023
The future is bright as technology continues to advance more rapidly than anyone can predict. AI leads the way as countries around the world strive to become proficient and find the next breakthroughs. Meanwhile, startups are looking to the stars as dreams of manufacturing higher-quality products in space inch closer to reality.
For the components industry, these developments are part of a larger trend of adaptation. As technology dictates how the world moves forward, the need for components is evolving but always present. Finding innovative ways to meet the demands of companies and countries pursuing advanced technology is paramount for years ahead. Â
U.K. Invests Millions in New AI Silicon but Eyes Chip Diplomacy as Path Forward
As the world turns its focus toward the exciting future of artificial intelligence (AI), every nation is racing to strengthen its digital capabilities. The U.K. recently announced an initiative that will see roughly $126 million poured into AI chips from AMD, Intel, and Nvidia. This move comes as part of a pledge made earlier this year to invest over $1.25 billion to bolster its domestic chip industry over the next 10 years. Â
However, critics of the move claim the government isn’t investing enough compared to other nations. Indeed, the U.K.’s latest investment is minuscule compared to those made elsewhere. The U.S. has invested $50 billion in its domestic semiconductor industry through the CHIPS Act while the E.U. has invested some $54 billion. Even so, the scope of the U.K.’s recent move shouldn’t be surprising, given that it accounts for just 0.5% of global semiconductor sales. Â
The recent injection of taxpayer money will be used to build a national AI resource. This will give AI researchers access to powerful computing resources and high-quality data to advance their work and the field. Other countries, including the U.S., are establishing similar programs to further their domestic AI capabilities. Â
The primary line item of the U.K.’s recent investment is reportedly an order of 5,000 GPUs from Nvidia, which are used to power generative AI data centers and are essential to running the complex calculations demanded by AI applications. The U.K. government is reportedly in advanced talks with Nvidia to secure these chips amid the company’s massive surge in international demand. Â
U.K. Prime Minister Rishi Sunak notes that Britain will focus on playing to its strengths rather than delving too far into areas where it is outmatched. For instance, the U.K. will devote a significant portion of its chip resources to research and design rather than building new fabs like many of its European neighbors. Â
Moreover, the U.K. seems poised to put itself in the center of the raging discussion surrounding AI safety. It recently announced that a long-awaited and highly publicized international AI safety summit will take place early this November. The meeting will include officials from “like-minded” governments as well as researchers and tech leaders. The group will convene at the historic Bletchley Park between Oxford and Cambridge, home of the National Museum of Computing and the birthplace of the first digital computer. Â
Interestingly, as the U.K.’s small investment compared to other nations will likely hinder its domestic chip ambitions, leadership in the regulatory space could be a fitting role. Britain reportedly aims to be a bridge between the U.S. and China for tense chip and AI safety discussions.
In a statement, a government spokesperson said, “We are committed to supporting a thriving environment for compute in the U.K. which maintains our position as a global leader across science, innovation, and technology.” Â
Meanwhile, China is racing to buy billions of dollars of GPU chips to further its own AI ambitions ahead of U.S. bans slated to go into effect in early 2024. At this time, it’s unclear if the U.K. will invite China to participate in its upcoming summit. Â
This will be an important development to watch as the U.K. aims to secure its position as a chip leader despite investing far less than other nations. While the U.S., Japan, Taiwan, and South Korea continue to dominate manufacturing, Britain could play a vital role in the future of the industry as a global moderator and champion of regulatory discussions.
How Manufacturing Chips and Drugs in Space Could Revolutionize Life on Earth
Outer space presents an environment for unique experiments that are simply impossible to perform on Earth. Astronauts living aboard the International Space Station (ISS) have been conducting such research for years. More recently, though, interest in manufacturing products in outer space has taken off. Â
From new pharmaceuticals to pure materials for semiconductors, the possibilities are endless. As a result, experts believe the space manufacturing industry could top $10 billion as soon as 2030. Startups and governments alike are racing to push the limits of this sector.
Manufacturing certain products on Earth, especially at a microscopic scale, is limited by factors like gravity and the difficulty of producing a reliable vacuum. In space, high radiation levels, microgravity, and a near vacuumless environment give researchers ample opportunity to produce materials or use research methods not available on Earth. Â
A Wales-based startup called Space Forge aims to revolutionize chip manufacturing by taking it into orbit. The company’s ForgeStar reusable satellite is designed to create advanced materials in space and return them safely to Earth. Â
Since crystals grown in space are of far higher quality than those grown on Earth, producing semiconductor materials in space leads to a final product with fewer imperfections. Andrew Parlock, Space Forge’s managing director of U.S. operations, said in an interview, “This next generation of materials is going to allow us to create an efficiency that we’ve never seen before. We’re talking about 10 to 100x improvement in semiconductor performance.” Â
The startup plans to manufacture chip substrates using materials other than silicon. In theory, this could lead to chips that outperform anything the world has seen to date while also running more efficiently. Â
As for concerns about manufacturing at scale, Space Forge CEO Josh Western says, “Once we’ve created these crystals in space, we can bring them back down to the ground and we can effectively replicate that growth on Earth. So, we don’t need to go to space countless times to build up pretty good scale operating with our fab partners and customers on the ground.” Â
As the semiconductor industry seeks new ways to make chips more efficient with current manufacturing technology nearing its limits, new materials made in space could be the answer. Though many years of research and testing will be needed, space manufacturing is a promising path for chip companies to explore. Â
Meanwhile, manufacturing drugs in space has also caught the attention of investors and researchers alike. Varda Space Industries relies on the unique ability to research and produce high-quality proteins in space through crystallization. This allows scientists to better understand a protein’s crystal structure so they can optimize drugs to be more effective, resilient, and have fewer side effects. Â
Varda co-founder and president Delian Asparouhov says, “You’re not going to see us making penicillin or ibuprofen… but there is a wide set of drugs that do billions and billions of dollars a year of revenue that actively fit within the manufacturing size that we can do.” Â
He notes that of all the millions of doses of the Pfizer COVID-19 vaccine given in 2021 and 2022, “the actual total amount of consumable primary pharmaceutical ingredient of the actual crystalline mRNA, it was effectively less than two milk gallon jugs.” Â
Once again, this alleviates concerns of producing drugs in space at scale. Rather than making the entire drug in space, companies like Varda will focus on making the most important components. Then, they’ll ship those back to Earth to complete the manufacturing process. Â
Thanks to recent advancements in spaceflight technology, such as reusable rockets, making missions to orbit cheaper, dreams of in-space manufacturing are inching closer to reality. Advancements in the coming years will help set the groundwork for what could be the new normal for manufacturing, one that allows humanity to go beyond the limits of what we can create on our planet.
‍

AI is Running the Chip Industry – August 25, 2023
No technology currently has a greater influence on the semiconductor industry than artificial intelligence (AI). From generative models like ChatGPT to massive data centers powering in-house algorithms, AI has sent demand for high-end silicon through the roof.
With demand soaring, chipmakers are scrambling to keep up and expand their production capacities. Cutting-edge AI solutions demand high levels of performance and optimized power efficiency. So, churning out advanced chips is a top priority for manufacturers across segments. Some chipmakers are even turning to an unlikely source for answers to stringent design challenges for future chips—the AI algorithms themselves.
As the chip industry grapples with the possibilities and limitations of AI, the technology’s influence is already redefining the landscape. Â
AMD Assures Production Capacity for Key AI Chips Despite Tight Market
AMD CEO Lisa Su had reassuring words for analysts and investors during the company’s second-quarter earnings call. Amid a booming market for AI chips, Su admitted that production capacity is tight, but that her company is poised to meet demand following numerous discussions with supply chain partners in Asia earlier this year. Â
She said in the earnings report, “Our AI engagements increased by more than seven times in the quarter as multiple customers initiated or expanded programs supporting future deployments of Instinct accelerators at scale.” Â
Large language models (LLMs) like ChatGPT have brought AI, particularly generative AI, to the forefront of the public’s eye. Companies in every sector are scrambling to get their hands on the necessary tech to keep up. For AMD, the generative AI application market and data centers are key strategic focal points. Â
With many AMD customers reportedly interested in the MI300x, it’s no surprise they hope to deploy the solution as soon as possible—despite the fact that AMD’s M-series GPUs were announced just a month ago. In the meantime, AMD is working closely with its buyers to ensure joint design progress and those deployments go smoothly as it begins sampling the new line. Â
Su noted in the Q2 earnings call that AMD has secured the necessary production capacity to manufacture its MI300-level GPUs—including front-end wafer manufacturing to back-end packaging. The firm is committed to taking in the massive capacity of neighboring supply chains, including TSMC’s high-bandwidth memory (HBM) and CoWoS advanced packaging. Over the next two years, AMD also plans to rapidly scale up its production capacity to meet soaring customer demand for AI hardware. Â
In the earnings report, Su said, “We made strong progress meeting key hardware and software milestones to address the growing customer pull for our data center AI solutions and are on track to launch and ramp production of MI300 accelerators in the fourth quarter.” Â
AMD plans to begin early deployments of the M-series GPUs in the first half of 2024. More rollouts will happen throughout the latter half of the year as a higher volume of M1300 units becomes available. Â
Importantly, Su also commented on the AI chip sector’s stiff competition, citing Nvidia’s market domination and Intel’s recent successes. She noted that the MI300’s flexible design allows it to handle both training and inference workloads. Notably, this capability is attractive to customers across multiple segments, including supercomputing, AI, and LLMs, giving AMD an opportunity to succeed in all these markets. Â
Simply put, Nvidia’s head start in the AI market won’t last forever. Although the chipmaker is significantly far ahead of everyone right now, it won’t be the only major supplier of AI chips in the long run. AMD is well-positioned to grab a significant chunk of the market and succeed alongside its competitors with its forthcoming MI300 series. With reassurances of secured production capacity in a tight market, we’ll likely see these chips in the real world sooner than later. Â
Researchers Are Using AI to Optimize Chip Design. But What Comes Next?
Computers designing themselves sounds like something from a science fiction movie. But it’s already happening inside the offices of Google’s AI-focused DeepMind, where researchers are using AI algorithms to solve chip design problems. Â
For years, experts have predicted that the end of Moore’s Law is near as chips continue to shrink and layouts become more complicated. In September 2022, Nvidia CEO Jensen Huang even declared the decades-old adage dead. Thanks to AI, though, the concept of doubling a chip’s transistor count every other year could get a breath of new life. Â
In a recent blog post, DeepMind researchers discussed how they are using AI to accelerate chip design. The novel approach treats chip design like a neural network, which consists of a series of interconnected nodes bridging the gap between inputs and outputs on either edge. To translate this into chip design, the DeepMind team created, “a new type of neural network which turns edges into wires and nodes into logic gates, and learns how to connect them together.” Â
The result? Circuits optimized for speed, energy efficiency, and size. Using this method, the team won the IWLS 2023 Programming Contest, finding the ideal solution to 82% of circuit design problems during the challenge. By later adding a reward function to reinforce the algorithm for positive design decisions, the team has seen “very promising results for building a future of even more advanced computer chips.” Â
It seems DeepMind researchers foresaw the future when they wrote in a 2021 paper, “Our method has the potential to save thousands of hours of human effort for each new generation.” Â
While AI isn’t ready to start designing chips all on its own, the promising results speak volumes about this vastly underexplored technique. Semiconductor leaders like Nvidia and Samsung are already using reinforcement learning algorithms to maximize the efficiency of their chips. Numerous startups are also exploring their own methods for using the power of AI to optimize semiconductor layouts. Â
However, it’s unclear whether the latest AI craze—generative AI—will play a role. Several companies and researchers are exploring how the technology could be used to optimize chip design, but analysts are doubtful. Â
Gartner VP analyst Gaurav Gupta said in a recent interview regarding the use of generative AI, “There is very limited evidence that I have seen so far.” Â
This partially calls back to the larger issue in the generative AI space of who owns what. Generative AI models like ChatGPT are trained on massive datasets to gain their skills. That data comes from internet sources—as does the data for most generic AI applications. But whether the resulting models can be used to create new designs that are then considered proprietary is uncertain. Earlier this week, a U.S. district judge ruled that works of art created by AI cannot be copyrighted. While not applying to semiconductors, this ruling could set an important precedent for other industries moving forward as the world grapples with how to regulate AI. Â
Even so, experts believe generative AI could still have a place in chip design—just not creating designs from scratch. The technology could be used to augment human-made designs or identify new ways to make a circuit layout more efficient. For now, though, it appears reinforcement learning algorithms will lead the way. Â
Over the coming years, more chipmakers will join the fray, making AI chip design more commonplace. As designs continue to shrink, AI will be a powerful tool for the industry to use to continue innovating and improving semiconductor performance and efficiency. Moreover, as demand for more capable chips to power AI applications grows, the technology could become a bit of a self-fulfilling prophecy, as older iterations are used to improve future functionality.
‍

Artificial Intelligence Continues to Attract Chipmakers - August 14, 2023
Original component manufacturers (OCMs) and semiconductor equipment manufacturers are working overtime to prepare for the incoming tidal wave of artificial intelligence (AI) demand. Â
The world has quickly fallen head-over-heels for the capabilities of AI. The popular generative AI model, ChatGPT, has been a trailblazer within the AI market, inspiring numerous copycat programs from big and small technology companies. As the competition heats up with ChatGPT equivalents, the need for higher computing power will become a top priority.
After all, these chatbots won’t be able to generate anything without these chips.  ‍
An AI Equipment Boom is on the Way
Artificial intelligence applications have exploded in use since the introduction of ChatGPT. Impressed with the capabilities of generative AI, the market sector is rapidly growing. With consumer demand and competition on the rise, semiconductor and chipmaking equipment maker Tokyo Electron sees a boom in equipment sales on the horizon. Â
The semiconductor industry has been experiencing a significant glut of excess electronic component inventory. When consumer demand wilted from increased prices due to inflation and high energy costs, there were worries over how long it would take for the industry to recover. To the surprise and delight of many generative AI, which quickly captured worldwide attention, will be the driving force of glut recovery. Â
With this explosion of popularity, the leading generative AI application ChatGPT is already driving growth within the semiconductor manufacturing equipment market sectors. The current impact is still relatively minor, but Tokyo Electron expects that to blossom into significant gains come 1H2024.
The semiconductor industry beyond equipment manufacturers will likely receive a significant boost in demand as technology companies and manufacturers work to dethrone the king of AI chips, Nvidia. Â
Tokyo Electron’s senior vice president Hiroshi Kawamoto said OCMs are already contacting the company regarding its GPU-making equipment product lines. Kawamoto told Nikkei Asia reporters, “The trend is still limited in scope, but I think we will start seeing a difference in revenue through April - September of 2024.”
“The number of semiconductors needed for generative AI servers will likely increase,” Kawamoto continued. “This technology could become our next driver for growth.”
As of late, like many OCMs, Tokyo Electron has faced a steady decline in demand during 2023’s chip glut. Demand for memory chip-related equipment has faced the steepest declines to no one’s surprise. Kawamoto doesn’t believe that will last for much longer. Â
“DRAM-related equipment will start looking up as early as the end of 2023,” Kawamoto said. “A real rebound won’t start until the next fiscal year.” He expects steady growth over the next fiscal year and forward due to the wide range of end uses for mature semiconductor manufacturing equipment, primarily utilized in automotive and industrial sectors. These industries have less volatility than their advanced node counterparts, as they can be subject to more extreme market shifts.
This is good news for the semiconductor industry, which is currently entering the peak period of excess electronic component inventory. It means faster recovery and mitigation of excess through selling before it costs manufacturers more by storing it correctly.  ‍
Chinese GPU Suppliers Eye AI Market
As semiconductor equipment manufacturers prepare for the oncoming AI equipment boom, so are graphic processing unit (GPU) suppliers. Many of which are eager to grab a portion of Nvidia’s expansive market share within the AI sector. Â
Artificial intelligence applications, inferences, and other programs require a lot of computing power. ChatGPT’s current model, GPT-3, runs 175 billion parameters to function. AMD’s latest chip, MI300X, only runs 40 billion parameters. Large-scale AI programs need a lot of high-performance GPUs to run significant tasks like content generation. Â
GPUs are the secret sauce behind many large language models (LLMs) like ChatGPT and other popular AI tools. With the sudden rise in popularity, GPUs have sprung into high demand overnight. Nvidia dominates, but OCMs of all shapes and sizes are working overtime to grab AI market dividends. Â
Chinese GPU suppliers and startups are no different. More so now that harsh sanctions by the U.S. are seeking to limit China’s access to new AI-capable electronic components. Â
The Shanghai-based startup, Denglin, has received funding and support from the China Internet Investment Fund (CIIF), the State Cyberspace Administration of China, and the Ministry of Finance to develop CUDA and OpenCL-compatible chips. CUDA is the name of Nvidia’s software package, allowing users to access the chip’s hardware features for development options. This software has been extremely popular among U.S. developers. Â
The chips Denglin has been funded to develop are reportedly used primarily for HPC and AI markets with their GPGPY usage capabilities. Denglin has announced four products this year, that are used for gaming and AI training. Its most popular solution is the Goldwasser chip. The chip is “designed for AI-based compute acceleration and will be getting edge and cloud gaming platforms.” Â
According to Denglin, its GPUs have been in high demand since last year when its 2nd generation GPU production capacity was completely booked. This new GPU will likely face the same high demand as it promises to deliver a 3 to 5 times greater performance gain for transformer-based models. Likewise, it is reported to greatly reduce hardware costs for ChatGPT and generative AI workloads, making it strong competition for Nvidia.
Predictably, a GPU supplier that offers the same CUDA and OpenCL capabilities for AI processing would be immensely popular in China’s domestic market. Especially now that restrictions are keeping Nvidia from making a splash within it. Nvidia GPUs’ hefty price tags are nothing to scoff at, either. Â
So far, thirteen other GPU developers within China are vying for the top spot. However, whether Denglin can prove popular enough to beat Nvidia on a global scale is far more uncertain. Â
‍
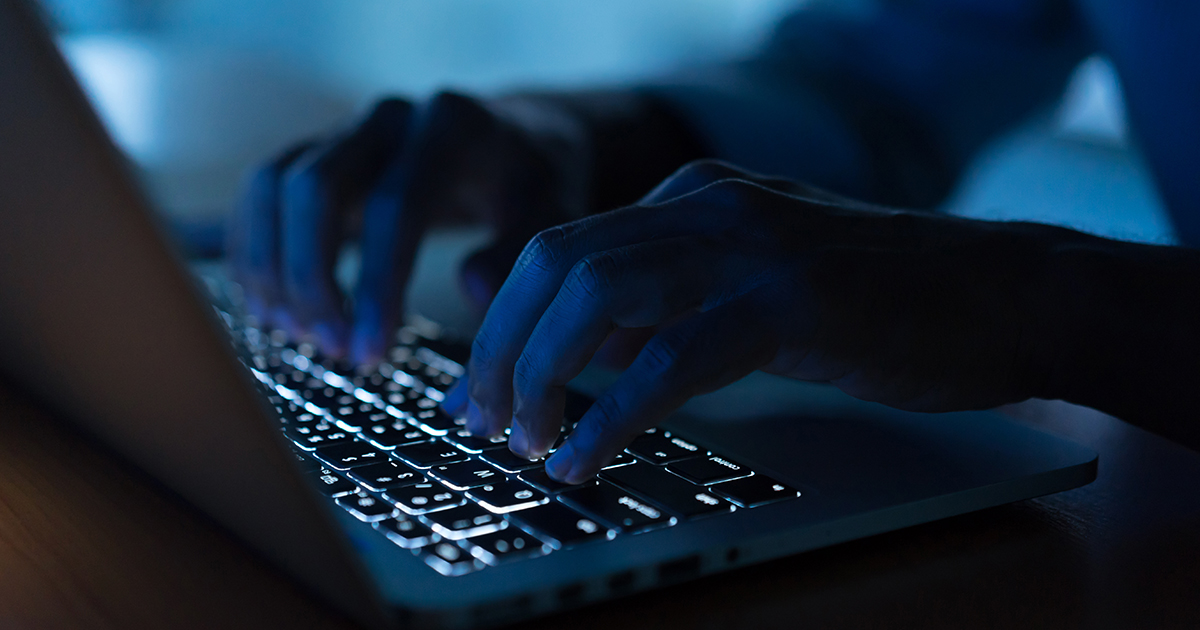
Supercomputers and China's AI Rules - July 28, 2023
The world of artificial intelligence (AI) is on an unstoppable upward trajectory of popularity and developmental breakthroughs. OpenAI’s ChatGPT lit the fuse within generative artificial intelligence and other AI applications. Now, tech companies and chipmakers across the board are working hard to develop their own generative AI chatbot or AI-capable components respectively.
With these discoveries come concerns. Industry leaders, including Elon Musk, are warning others of the potential dangers of unrestricted artificial intelligence. Equally concerned are government lawmakers who are now beginning to draft the first set of laws to safeguard the public from AI-generated misinformation. Â
Tesla’s Upcoming Supercomputer Entering Production
Earlier this year, Tesla CEO Elon Musk called for a pause on artificial intelligence, citing concerns that mismanaged design could lead to “civilization destruction.” Musk’s critiques of artificial intelligence are nothing new. Musk’s contradictory stance on artificial intelligence, condemning it publicly while increasing AI development within his companies has been well-known for years. Â
When Musk learned of OpenAI’s and Twitter’s relationship, where ChatGPT was built on user Tweets, Musk severed ties. Formerly, Musk helped found the AI lab in 2015.
Recently, Musk has continued expressing his concerns on artificial intelligence and is reported to be working alongside Microsoft's CEO in approaching the EU to discuss the best strategies for AI regulations. Â
Meanwhile, Tesla’s AI team recently announced on Twitter the ongoing progress of Tesla’s upcoming custom supercomputer platform Dojo. According to the tweet, the computer will go into production in July 2023 and is expected to be “one of the world’s five most advanced supercomputers by early 2024.” Â
Reports by Electrek and Teslarati detail Dojo’s progress as another significant step by Tesla to carve out a spot for the company within the AI market. Furthermore, Tesla is working to reduce its dependence on traditional chipmakers, like Nvidia, whose A100 and H100 GPU chips are dominant within AI applications, which is true for even some Tesla AI products. In contrast, Dojo uses AI machine learning that utilizes Tesla-designed chips and infrastructure which are trained on data from Tesla's feed to develop its neural network.
After Dojo’s launch in 2021, the supercomputer has continued to be developed over the last few years with the goal of supporting Tesla’s vision technology to obtain complete autonomous driving. Musk has been working hard to make this area of AI a reality. Despite his critiques of other AI developments, Musk is pleased with the progression and advancements by the Tesla AI team in both software and hardware. Â
Dojo is expected to be Tesla’s first step in creating a powerful computing center capable of handling many AI tasks. Tesla currently uses Nvidia’s GPUs in its previous supercomputer to process FSD autonomous driving data. Dojo should be able to process more video data, contributing to the acceleration of iterative computing in Tesla’s Autopilot and FSD systems. Eventually, Dojo could be able to provide the significant computing power required for Tesla’s other projects, including its humanoid robot Optimus.
Dojo’s supercomputers will greatly aid Tesla’s growing workload as the company strives for independence by designing its own chips and AI applications. With the data-driven insights provided by Dojo through Tesla’s video data, Tesla could come closer to making autonomous driving a success. By extension, the industries that could benefit from Dojo’s insights would be extensive, especially in a more interconnected world. Â
However, it will be interesting to see how upcoming AI regulatory efforts impact the later development within the market.  ‍
China is First to Begin Major AI Regulation
Microsoft and Tesla leaders are heading to the EU to discuss the need for tech leaders to be involved in establishing AI regulation. In comparison, China has moved ahead in establishing new requirements for generative AI. As one of the first countries to regulate generative AI used in popular tools like ChatGPT, other countries might use their laws to guide their regulations. Â
The Cyberspace Administration of China (CAC) recently announced updated guidelines for the growing generative AI industry. These new rules are set to take effect on August 15th, and it appears in this “interim measures” document several previously announced provisions have been relaxed. Â
The announcement comes after regulators fined fintech giant Ant Group a little under $1 billion. This fine followed a series of regulatory strikes against other tech giants, including Alibaba and Baidu, who are all launching their own AI chatbots, much like Microsoft and Google. Â
The new regulations will apply only to services available to China’s general public. Technology developed in research institutions or created for users outside of China are now exempt. The language that indicated punitive measures, including hefty fines, had been removed not to limit AI's ongoing development within China. Â
In the document, the CAC “encourages the innovative use of generative AI in all industries and fields by supporting the development of secure and trustworthy chips, software, tools, computing power, and data sources.” Beijing urged tech platforms to “participate in formulating international rules and standards” regarding generative AI. A regulatory body would aid in continued monitoring and culpability to hold each other accountable. Â
It will be interesting to see how other countries will follow suit, especially now that tech leaders are courting lawmakers to collaborate on AI regulations. Going forward, it will be pertinent for tech companies and governments to work together to form a robust and flexible foundation to encourage AI development, not hinder it, and safeguard users. Â
‍

AI Rules and Regulations on Big Tech’s Mind - July 14, 2023
In May 2023, a few months after the massive boom of OpenAI’s ChatGPT, Geoffery Hinton, dubbed “the Godfather of AI,” quit Google. With his departure came a warning that artificial intelligence could soon grow smarter and possibly manipulate humans. Unfortunately, most of the general public saw those concerns and thought of AI turning on its human creators and overrunning the world with an army of metallic soldiers. Â
No, that’s not what Hinton and other tech leaders like Microsoft’s Brad Smith are concerned about with artificial intelligence (AI.)
In an interview with CNN, Hinton discussed concerns about current challenges and rising problems within AI becoming more notable with its fast developments. Specifically, Hinton’s immediate concerns are that “the internet will soon be flooded with fake text, pictures, and videos that regular people won’t be able to distinguish from reality. This could, he said, be used by humans to sway public opinion in wars or elections. He believes that A.I. could sidestep its restrictions and begin manipulating people to do what it wants by learning how humans manipulate others.”
These concerns are not unfounded. Presently, some natural language processing chatbots hallucinate or deliver users false information. In many AI-generated images, artists have expressed concern over being replaced or having their work copied and regurgitated by AI faster and cheaper. However, far-reaching and general restrictions will not fix the issues with AI that concern Hinton and other AI critics. Â
Better monitoring through regulations based on conversations between lawmakers and tech leaders will be vital in establishing guidelines to prevent the spread of false AI information. Â
Microsoft President Discusses AI Regulation with the EU
Artificial intelligence has been making headlines ever since OpenAI’s ChatGPT came out. With artificial intelligence’s rise, so too have the chips that make these AI feats possible. As the possibilities within AI, specifically generative AI, are explored, the benefits have been marred by growing concerns. Â
Attention-grabbing headlines calling the rise of AI the doom of humanity explore the idea of artificially intelligent automatons enslaving their human creators are more fiction than fact. However, for every dystopian sci-fi article that dramatizes the dangers of AI, there is an article that rightly discusses the concerns about the growing use of artificial intelligence, mainly regarding areas where AI still lacks. Â
Artificial intelligence is, without a doubt, a fantastic tool. The capabilities of AI will be pertinent for all organizations in the next cycle of growth to aid the work and development of human staff. Automation, predictive analytics, market intelligence, generative AI, and other developments will help companies refine processes, increase operational efficiency, and improve employee morale. Â
That said, AI is not perfect. On debut, Google’s Bard AI made a factual error about the James Webb Telescope, and Microsoft’s Bing AI created fictional financial information. These specific errors are part of an inherent problem with AI called artificial hallucination. These hallucinations are “generated content nonsensical or unfaithful to the provided source content." There are several reasons why or what causes an AI to hallucinate. In natural language processing models like Bing, Bard, and ChatGPT, the cause of hallucinations mostly centers on divergence from the training source material or filling in gaps that were not within the training data. Â
The biggest concern is for internet-data scouring large language models, which is that the internet is full of false information. Information that trains and feeds many of these language models AI bots. Â
Brad Smith, Microsoft’s president, believes that regulating artificial intelligence can benefit AI development. Alongside Tesla CEO Elon Musk, Smith is courting regulators and lawmakers with calls to regulate AI and how big tech companies, like Microsoft and Musk’s Twitter, can help refine the guidelines. Â
The timing is perfect as the European Union works out the rules for its coming AI Act, which could set a benchmark for other countries to follow. Â
For many countries, it is well-known that many lawmakers are exceedingly tech illiterate. Earlier this year in the U.S., the world watched in silent awe as Congress grilled TikTok CEO Shou Zi Chew for hours. More recently, many waited with bated breath as the U.S. Supreme Court ruled two cases against Google and Twitter, respectively, which many believed had dire implications for the future of AI. The U.S. Supreme Court ruled in favor of Google and Twitter. Â
Having tech giants work alongside lawmakers to create AI regulations would help both parties create a strong but fair system. With big tech’s help, lawmakers could better understand and pass requirements that are not too draconian and inflexible but stringent to protect the public.
"Our intention is to offer constructive contributions to help inform the work ahead," Smith said. He addressed Microsoft's five-point blueprint for governing AI, which includes government-led AI safety frameworks, safety brakes for AI systems that control critical infrastructure, and ensuring academic access to AI aligns with the EU's proposed legislation.
Furthermore, Smith urged the EU, the United States, G7 countries, India, and Indonesia to work together on AI governance in line with their shared values and principles. With AI growing in use, hopefully, further collaboration between countries will make a standardized set of requirements, making it far easier for AI developers to follow. Â
Digitalization Optimizes Manufacturing for Modern-Day Demand
Experts have said digital transformation is critical to an organization’s success in an increasingly tech-integrated world. Utilizing digital technologies in existing processes increases efficiency, agility, and profitability. The smoother your operations run, the higher customer satisfaction becomes. Staying competitive today requires digitalization.
In an article by Forbes, data from an L2L survey via Plant Engineering found that 24% of manufacturing companies have a digital strategy. Out of those companies, over 40% have yet to take the first step to begin implementation. This is especially surprising when one considers that manufacturing stands to benefit the most from digitalization. Â
According to the L2L survey, one of the biggest struggles that prevent organizations from initiating a digital transformation is moving away from decades-old legacy systems. Especially since the old adage often rings true, “If it ain’t broke, don’t fix it,” right? Except, these legacy systems contribute to the same challenges that feed the flames of chip shortages and other disruptions. Â
Unfortunately, manual systems are more prone to errors or inaccuracies than digitalized processes. The leading cause is human error. This is especially true in procurement for electronic components due to the extensive information stored on Excel sheets. With AI and other digital tools, this information is stored instantly and, most importantly, accurately. Â
Increased amounts of accurate data provide better supply chain visibility. With these data-driven insights, organizations can make more informed decision-making faster without wasting the most valuable commodity within any industry; time. Better visibility gathered through digitalization can give a detailed picture of market opportunities, allowing companies to optimize manufacturing processes and beat competitors by large margins. Â
In 2020, a Deloitte survey found that “greater levels of digital maturity are major drivers for annual net revenue growth up to three times higher than competitors with a less-developed digital transformation strategy.” According to Microsoft’s 2019 study on Tech Intensity, most leaders found that among 700 executives, 75% believed harnessing tech intensity, the rate of tech adoption, and capability is the most effective way to build a competitive advantage today.
That percentage is only increasing. In a Forbes article, research states, “Digitalization is the best way to boost productivity, production quality, revenue growth, hiring appeal, and future market share.” The longer organizations rely on legacy systems. The further the gap grows between those that have digitalized and those that haven’t. A digital transformation will only become more complex and costly for those not taking the first step soon. Â
One of the simplest ways to begin their digital transformation is by utilizing a digital market intelligence tool such as Datalynq. Upon signing up for a free trial, Datalynq can instantly deliver data-driven insights on the electronic component supply chain. Find out how to strategically prepare and create a more resilient supply chain today. Â
‍
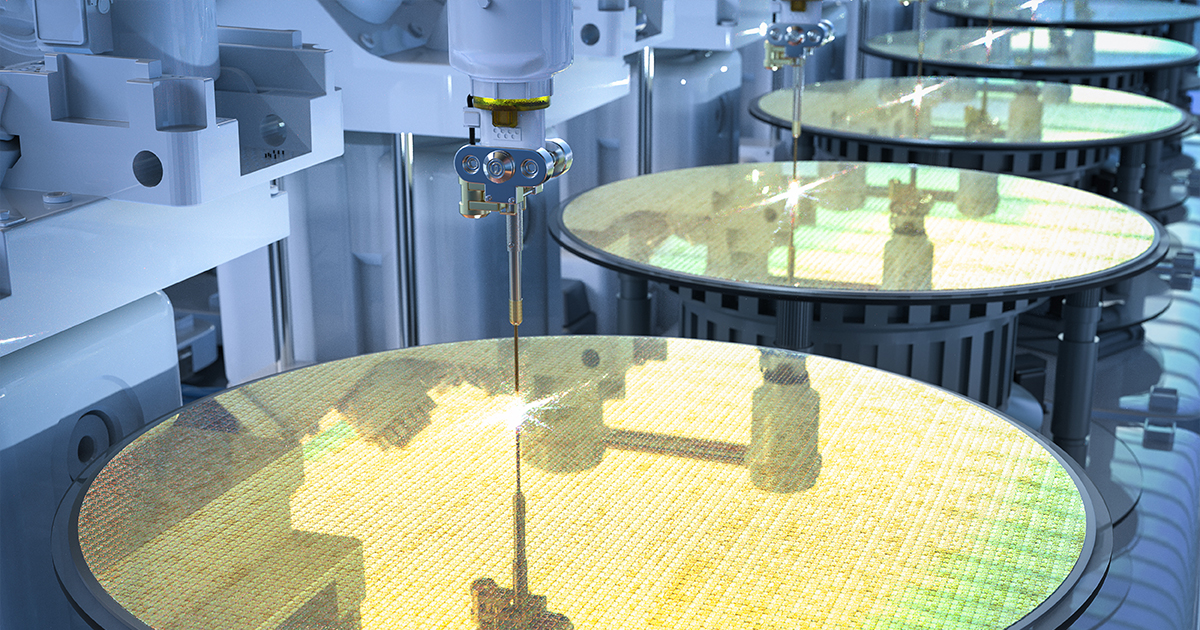
AI is Redefining Chip Making - June 30, 2023
Artificial intelligence is changing the way we work. No, AI isn’t here to replace us or take our jobs. AI still has a long way to go before it can accomplish the complex thought process of the human mind, despite recent leaps and strides. Artificial intelligence is, however, a magnificent assistant. Â
Nvidia’s GPUs are powering some of the most fascinating advances within the field of artificial intelligence, specifically in generative AI. Currently, Nvidia stands alone in its dominance. The company’s GPUs have been swiftly gobbled up by tech giants such as Apple and Microsoft. Many chipmakers have quickly shifted gears to focus on producing their own coveted AI chips, none have gotten close enough to pose a challenge. Until now, that is. Â
AMD Challenging Nvidia with New Chip
Nvidia is currently secure as the king of artificial intelligence chips, but it might not be that way for long. Ever since OpenAI’s ChatGPT kicked off the latest artificial intelligence revolution, chipmakers have been seeking a way to take Nvidia’s crown. Among these upcoming competitors is AMD and its latest AI-capable chip. Â
According to experts, it might be the strongest competition Nvidia has yet.
It’s called the MI300X, and AMD says it's the most advanced GPU for artificial intelligence that will become available later this year. Nvidia dominates the market for AI chips with over 80% of the market share due to their use by modern AI firms such as OpenAI. This new chip could steal some of that audience. Â
Traditionally, AMD is known for its computer processors. However, AMD CEO Lisa Su believes that AI could be the “largest and most strategic long-term growth opportunity” for the company. Tapping into the AI market could help AMD see similar growth to Nvidia. Su believes that from 2023 to 2027, the AI market could see over a 50% compound annual growth rate with its current pace. It’s no wonder chipmakers are eager to throw their hats in the ring.
In a statement on the MI300X, AMD said MI300X’s CDNA architecture was designed for large language models and other cutting-edge AI. Due to the popularity of GPUs within the subset of artificial intelligence called generative AI, the MI300X being outfitted for that particularity makes it a strong competitor to Nvidia’s GPUs. Â
This is seen especially in memory, as AMD’s MI300X can store up to 192GB compared to Nvidia’s H100, which only supports 120GB. This makes AMD’s chip one of the few that can fit in bigger AI models. This is especially worthwhile for large language models as these specific AI applications need extra memory for their algorithms' large number of calculations. Most large language models currently use multiple GPUs to achieve this. Â
During the MI300X’s announcement, the chip ran a 40 billion parameter model called Falcon. For comparison, the current ChatGPT model, GPT-3, has 175 billion parameters making numerous GPUs a given necessity. Like Nvidia and Google, AMD plans to offer Infinity Architecture, combining eight chips into one system, for AI applications. Likewise, it should come with its own software package AMD, called ROCm, similar to Nvidia’s CUDA. Â
This software would give AI developers the capabilities to enable the chip’s core hardware features, something many developers prefer. Â
As of now, there has been no word on what the cost of AMD’s chips will be. Nvidia’s popular GPUs can run upwards of $30,000, making them an expensive investment. If AMD’s MI300X comes with a smaller price tag but the same capabilities that endear Nvidia’s GPUs to developers, AMD will likely gain a large and excited audience. Â
Samsung Applies AI to Chip Manufacturing
Automating production lines is one of the simplest but most effective tools to both reduce human error and save on operation costs. Unsurprisingly, after the pandemic shut down semiconductor fabrication plants for weeks, many original component manufacturers (OCMs) have begun automating their lines. Samsung Electronics is no different. Â
Samsung Electronics is implementing AI and big data into its chipmaking process to enhance productivity and refine product quality. The president and head of Samsung’s semiconductor department, Kyung Kye-Hyun, is leading the charge to improve wafer manufacturing yields and catch up to foundry leader TSMC. Â
It might be the only way to get on an even playing field with TSMC, as sources say the chipmaker uses an AI-enhanced manufacturing method called AutoDMP, powered by Nvidia’s popular DGX H00 chips. This process will likely be used to produce TSMC’s upcoming 2nm process, as this AI-enhanced method optimizes chip designs 30 times faster than other techniques, something Samsung is eager to recreate. Â
In partnership with the Samsung Advanced Institute of Technology (SAIT), Samsung’s Device Solutions (DS) division, which oversees its semiconductor business, Kye-Hyun will lead the efforts to broaden and improve AI methods within their chip manufacturing process. According to those familiar with the matter, Samsung will specifically use AI for “DRAM design automation, chip material development, foundry yield improvement, mass production, and chip packaging.”
One of the benefits of utilizing AI that Samsung specifically targets is limiting and determining the source of unnecessary wafer losses while analyzing and removing DRAM product defects. Since AI excels in pattern recognition, it will be a considerable improvement to have AI assist in production lines to eliminate errors before they get too far. Â
AI is becoming more essential for chipmakers in ultra-fine processes as the narrower the circuit width on a wafer, the higher the chances of transistor interference and leakage current. Kye-Hyun said earlier this year that “the gap between companies that utilize AI and those that don’t will widen significantly.” Â
For smaller or mid-sized OEMs that do not have the bandwidth to optimize production lines with AI, there are other digital tools that can help streamline other processes so staff can devote more time to maintaining operations sufficiently. Market intelligence tools that utilize real-time market data and historical trends can aid manufacturers in strategizing for possible component obsolescence, future disruptions, and create more resilient BOMs. Datalynq is one such tool, and it offers a 7-day free trial for those ready to see how AI can help your organization succeed. Â
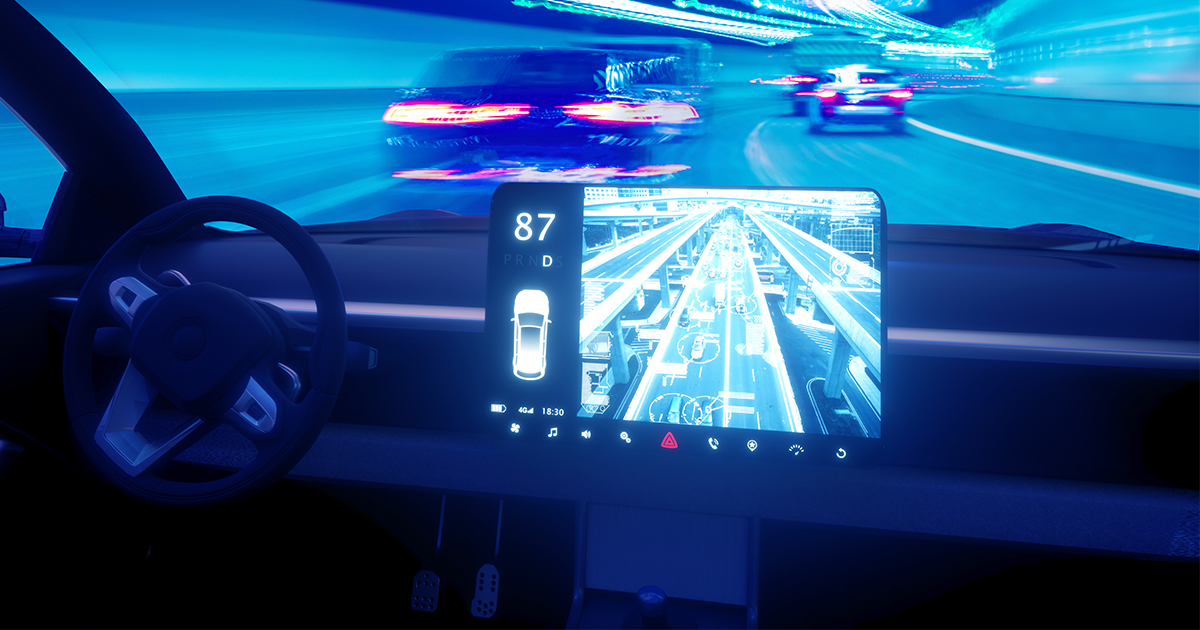
Artificial Intelligence is the Word - June 16, 2023
The bird isn’t the word anymore! Artificial intelligence (AI) is, and it continues taking the world by storm. While AI opens new avenues within business thanks to data-driven insights and other capabilities, AI is useful in dozens of applications outside traditional information technology solutions. One of those industries is automotive. Â
AI has been growing in use within the automotive industry for years and has only blossomed since the dream of a fully autonomous vehicle. The feasibility of such a vehicle might still be years away, but in the meantime, AI is set to boom within vehicles over the next ten years. With that growth, the industry expects to see new capabilities develop thanks to data attained by automotive AI. Â
First, however, the chips able to perform such feats must be created, and it doesn’t sound as if they are too far off. Â
AI Takes Centerstage at Computex
Computex Taipei, or Taipei International Information Technology Show, is an annual computer expo that’s taken place in Taiwan since the early 2000s. As one of the world's largest computer and technology trade shows, it continually spotlights new and emerging technology areas. Many of these evolve into great importance as they enter mass production. Â
Over the past few years, Computex has focused mainly on the evolution of PC and cloud-centric applications that support the Internet of Things (IoT) and artificial intelligence of things (AIoT). These applications have continued to amplify the importance of edge computing, prompting many organizations to catch up to the continued growth of the cloud. Â
With computing’s deep ties to electronics, it's no surprise that numerous semiconductor manufacturers also attend, including Arm, MediaTek, Cirrus Logic, and other small-to-medium IC design firms. This year, Nvidia took the lead front and center, with CEO Jensen Huang kicking off the show with a keynote speech. Â
Qualcomm gave its own speech, sharing its innovative new product lines for AI applications powered by its mighty Snapdragon chips. For eager audience members, Qualcomm once again teased its highly anticipated Oryon CPU, which was unveiled last year. The Oryon CPU is expected to increase performance and efficiency in AI applications for smartphones, digital cockpits, and advanced driver assistant systems (ADAS).
“AI technology is experiencing a remarkable boom, and the profound impact it has had on our daily lives is nothing short of astonishing,” Qualcomm said.
NXP Semiconductor announced a new processor, the i.MX 91 for industrial, medical, consumer, and IoT applications. The new processor is part of NXP’s product longevity program to ensure qualified products are available for at least ten years, a necessity for automotive, telecom, and medical markets. The i.MX 91 can help smart home devices communicate with one another, increasing the capabilities of emerging smart home technologies. Â
Texas Instruments shared its plans for the future of embedded processing and AI. TI’s VP for processors, Sam Wesson, said, "Based on what we see in the industry and what we hear from our customers, three trends stand out as crucial capabilities that embedded designers are looking for more integrated sensing capabilities, enabling edge AI in every embedded system; and ease of use so customers can get to market faster. At TI, we are innovating to enable new capabilities in embedded design while reducing system cost and complexity."
The emerging use of AI applications, especially generative AI such as large language models (LLM), has thrust AI into the spotlight of both the semiconductor industry and the world. Thanks to decades of investments in AI, Nvidia is currently leading the race in chips equipped to handle the growing demands of AI innovations. Computex proved that attention is now laser-focused on AI developments, and chipmakers are quickly following suit.
As other original component manufacturers (OCMs) begin their foray into AI, Nvidia continues to build its already impressive portfolio. After all, Nvidia’s growth will “largely be driven by data center, reflecting a steep increase in demand related to generative AI and large language models,” Colette Kress, Nvidia’s finance chief, said. OpenAI’s GPT-4 large language model, trained with Nvidia GPUs on extensive online data, is at the core of ChatGPT.
It’s expected, based on news shared at Computex, TI, NXP, STMicroelectronics, Nvidia, and others, will continue to increase their share within the AI marketspace with chips capable of handling AI’s impressive demands. Â
The Growing Use of AI in Automotive Applications
AI is growing in use in many industries. From smarter homes to radiography assistants detecting mammograms for breast cancer, artificial intelligence is expanding into any and all organizations. It should come as no surprise that AI within the automotive market, where chipmakers and automotive original equipment manufacturers (OEMs) alike are looking to produce fully autonomous vehicles, is booming. Â
The current market share is a respectable $9.3 billion for 2023. It is anticipated to set a robust CAGR of 55% and boom to a value of $744.39 billion by 2033. That is a significant amount of growth over ten years. The reason for this explosive increase in AI market share can be linked to autonomous vehicles, as expected. Â
ADAS and auto-driving modes are utilizing AI solutions to expand performance and efficiency within automotive technology. Incorporating AI has led to the transformation and creation of services such as guided park assist, lane locator, lane assist, and other technologies. Research and development programs have increased automotive AI sales as the automotive industry works to re-implement smart features after their cuts during the worst global chip shortage. Â
People like personalization. AI features satisfy the desire to customize an electronic that suits a user's needs and delivers ease of use for users. AI-enabled applications for autonomous operations are expanding the demand for AI-integrated automotive systems. As a result, automotive engineers designing new cars with newer and better operating systems that utilize AI will drive that explosive market growth. Â
AI machine learning integration within the automotive market is also helping engineers develop better systems based on data-driven insights gathered from drivers. This includes AI-integrated systems that observe driving patterns to aid in developing advanced guidance and assistance. An AI model that aids with this pattern recognition is through AI with machine learning and Natural Language Processing (NLP). The same type of AI that makes up the world’s new favorite chatbot, ChatGPT. Â
For the future of AI in automotive applications, it is hard to determine what the vehicles of tomorrow will look like today. Based on the current market trend, machine learning will become an integral part of vehicle production as it will significantly aid engineers in the future to make more sustainable and smarter technology. Many automakers, like Tesla, will strive to develop a truly autonomous vehicle requiring no human interaction. Â
Likewise, AI integration will only continue to flourish as electric vehicles (EVs) continue to rise in use thanks to government incentives and environmental measures. As it stands, EVs produce large amounts of data on their own. AI can help track, monitor, and report such data to users and manufacturers. Â
However, the more chips a vehicle uses, the more prone the industry becomes to shortages. Limited application of sensors and equipment within the vehicle would weaken AI and machine learning systems. Both need a lot of computing power to support them. Though, with countries passing incentive plans for semiconductor manufacturing, the supply of chips will increase as new facilities come online. Â
It is pertinent for OEMs and other manufacturers to monitor market conditions to strategize for possible shortages in advance, to prevent future production stalls from undersupply. Also, manufacturers forming partnerships with chipmakers to ensure production capacity is always assured no matter the year will help solidify supply and improve relationships through collaboration. The former can be accomplished with help from Datalynq’s market intelligence. Â
‍
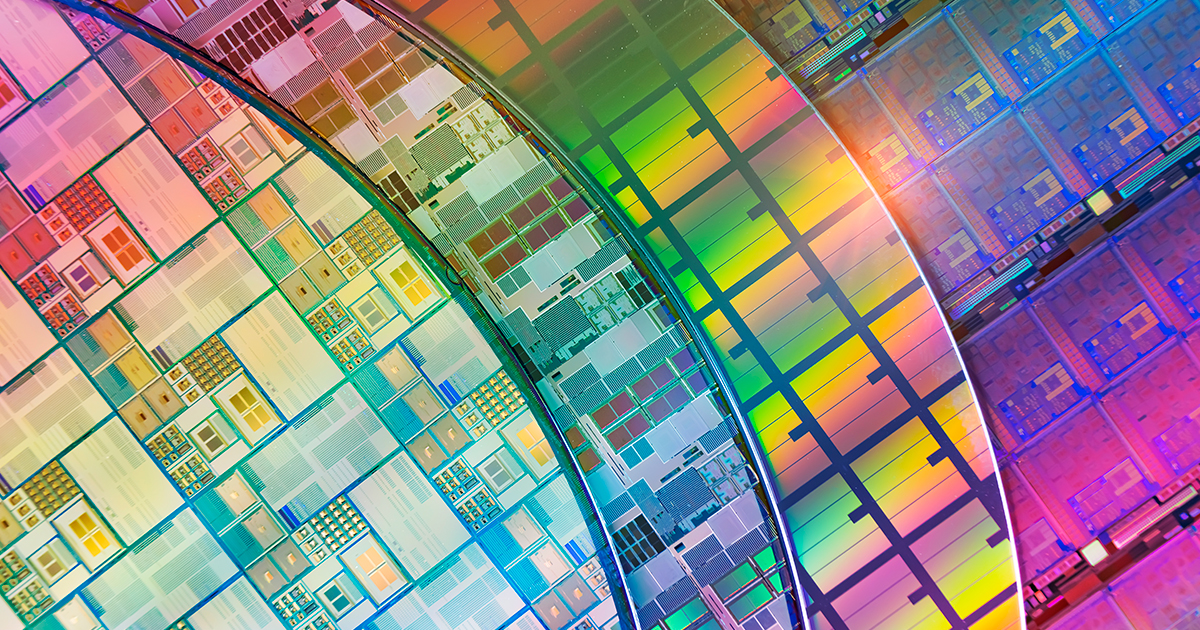
GPUs for AI and Semiconductor Manufacturing - June 2, 2023
The semiconductor market is recovering, but a recent trend might push one chip maker back to the beginning of the global chip shortage. Nvidia has been in the spotlight recently for its latest product debuts in artificial intelligence (AI) and its specific type of microprocessor has taken the world by storm for its benefits to AI applications. Â
As AI booms, so too does Nvidia’s products and, as the sole supplier of many of them, the more AI grows, the scarcer the supply becomes. If AI is going to be the one to bring us out of the current chip glut, it could turn out to be a very sharp double-edged sword. Â
However, the options are expanding in electric vehicles (EVs) as EV use grows. Japanese chipmaker Renesas announced their plans to begin production on their own silicon carbide (SiC) chips for EV applications. While it is not the only competitor within that market, its products will likely be a hit among many EV manufacturers. Â
Nvidia GPUs Are the Hot Ticket Item of the Year
Nvidia’s graphic processing units (GPUs) are powering a new evolution in technology and chip manufacturing. After years spent betting on the success of artificial intelligence, Nvidia’s long-term strategy is starting to pay off in part to GPUs. Â
GPUs are accelerating processes. CPUs dominated most workloads in both AI and manufacturing processes, but GPUs are specialized components that excel in running multiple tasks simultaneously. Â
GPUs are accelerating the same manufacturing process that creates them. TSMC, ASML Holding, and Synopsys have used Nvidia's accelerators to speed up or boost computational lithography. Meanwhile, KLA Group, Applied Materials, and Hitachi are now using deep-learning code running on Nvidia’s parallel-processing silicon for e-beam and optical wafer inspection.
In computation lithography, manufacturers etch chips into silicon by projecting specific wavelengths of light through a photomask. The smaller the transistor on silicon dies become, the more creative engineers have to be to prevent distortion from blurring those tiny features. These photomasks are so ornate they're generated on massive computer clusters that can take weeks to complete. Well, at least it used to be.
According to The Register, Nvidia's CEO Jensen Huang claims this process can be sped up by 50x by GPU acceleration. "Tens of thousands of CPU servers can be replaced by a few hundred DGX systems, reducing power and cost by an order of magnitude.”
But improving manufacturing processes has only been the tip of the GPU benefit iceberg. AI will become more dependent on high-performing GPUs to accomplish its many tasks as it booms. Most large-scale AI depends on GPUs to allow it to keep up with the numerous algorithms that it is comprised of. At the ITF semiconductor conference, Huang spoke of AI and GPUs' potential to breathe new life into Moore's Law. "Chip manufacturing is an ideal application for Nvidia accelerated and AI computing.” Â
Semiconductor manufacturing facilities are already highly automated, but Nvidia is branching out, using those tasks as a base, to move into robotics, autonomous vehicles, chatbots, and even chip manufacturing. Recently Huang teased a new product, VIMA, a multimodal "embodied AI model trained to perform tasks based on visual text prompts, like rearranging objects to match a scene…I look forward to physics-AI robotics and Omniverse-based digital twins helping to advance the future of chipmaking.”
Despite the downturn in chip demand, Nvidia is posed not only to come out relatively well-off but possibly unable to meet the growing demand. Especially now that large foundry operators, including Samsung Electronics, SK Hynix, Intel, and TSMC, are pushing ahead with new projects. New government incentives, such as the US and EU Chips Acts, have pushed forward new facility plans which, in turn, require more GPUs to automate the manufacturing and design processes via artificial intelligence and machine learning.
More facilities will likely use AI/ML going forward, primarily due to many countries' lack of skilled labor. To keep costs low and production high, automating numerous tasks for the small talent pool to oversee will be the path forward. Â
The AI boom brought on by ChatGPT has caused demand for high-computing GPUs to soar. DigiTimes reported that “Nvidia has seen a ramp-up in orders for its A100 and H100 AI GPUs, leading to an increase in wafer starts at TSMC, according to market sources. Coupled with the US ban on AI chip sales to China, major Chinese companies like Baidu are buying up Nvidia's AI GPUs.”
As a result, Nvidia components could experience a shortage. This would be incredibly impactful because Nvidia is currently the sole supplier of most AI-capable GPUs. The likelihood of this possibility continues to rise as OCMs increase their use of Nvidia’s GPUs. That is unlikely to stop because, as of now, Nvidia’s GPUs can even outperform a quantum computer. Â
Tracking lead times and availability will be a top priority to keep abreast of the shifting market and the possibility of Nvidia being affected by future shortages. Datalynq can help you plan.
Renesas To Begin Making SiC Chips for EVs
As Nvidia leads the charge in AI, Renesas is introducing new components for the growing EV industry. By 2025, Renesas will begin producing next-generation power SiC semiconductors. These new semiconductors will be manufactured in Takasaki in Japan's Gunma prefecture, northwest of Tokyo. Â
The site currently produces silicon wafers but will soon begin the shift later in the year. The SiC chips are expected to power the MOSFETs for EV inverters alongside a new gate driver IC Renesas announced in early January 2023. Compared to traditional silicon, SiC chips offer better heat resistance and reduced power loss, contributing to longer driving ranges. Â
Numerous chipmakers, including Infineon Technologies and STMicroelectronics, have explored SiC semiconductor capabilities over the last few years. Renesas is behind the curve in this area. Renesas President Hidetoshi Shibata isn’t too concerned with playing catchup, as the chip giant has done it once before. Â
Renesas "was a latecomer in conventional power semiconductors, but now [our products] are valued for their high efficiency," Shibata told reporters on Friday during the announcement. "The same can be done with SiC."
Despite Tesla’s 75% cut of SiC chip use in their future vehicle line-ups, the EV industry isn’t wavering in its use. SiC has proven itself to be a more efficient component within EV applications, and it's likely it will continue to be used in power-saving solutions for its benefits over traditional silicon. Especially now that silicon carbide is becoming more affordable as its manufacturing process is further refined.
‍
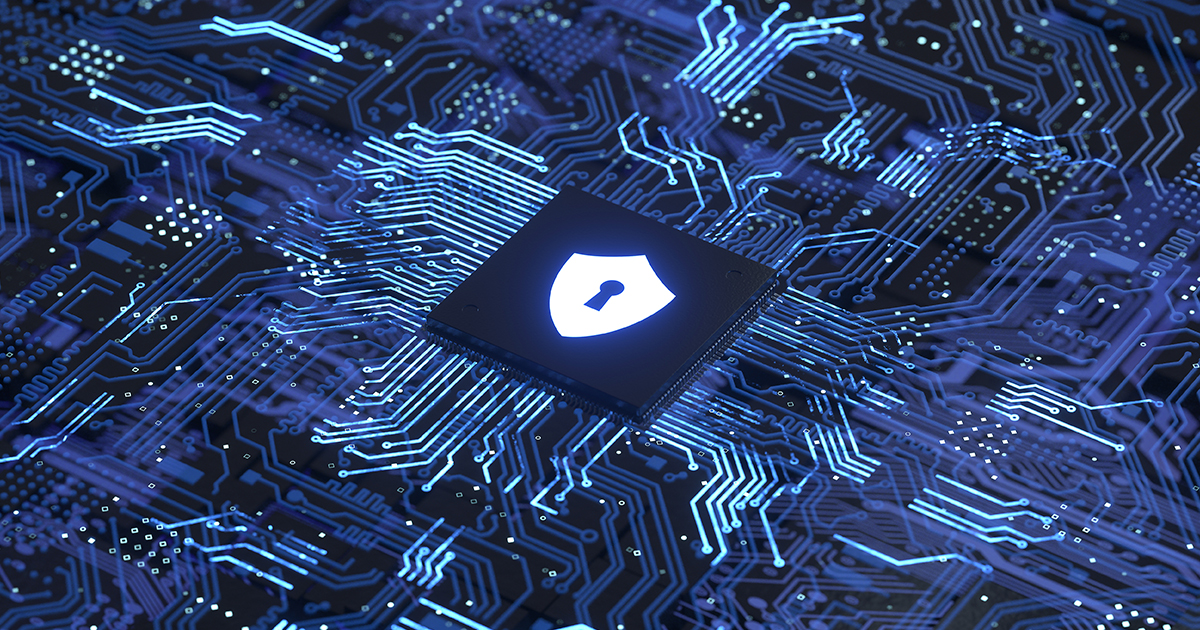
Lack of Digitalization Costs Companies Far More than Revenue - May 19, 2023
The digital age is upon us. However, many organizations are still unprepared to adapt. Financial losses are the last thing you must worry about for those who don’t upgrade in the ever-evolving digital landscape. Â
Leading the charge in technological developments is artificial intelligence (AI), thanks to recent AI platforms making waves. AI shouldn’t be viewed as a terrifying boogeyman out to replace humans but as a tool that helps make excellent staff perform even better. Chip giant Nvidia is one of the manufacturers leading the charge to help organizations of all sizes implement AI into their workflows. Â
Nvidia’s AI Tech is Transforming Enterprise Workflows
2023 is quickly becoming the year of AI. After OpenAI’s ChatGPT popularity boom in December, the last few months have been nothing but AI launch after launch. Experts believe that growing interest in AI technology will keep semiconductor demand up throughout 2023, with expectations it will ease the chip glut the industry is currently struggling with. Most of that recovery is forecasted to begin in late 2023. Â
While Google and Microsoft work to perfect their ChatGPT equivalents after some troublesome debuts, one original component manufacturer (OCM) is helping companies kick off their AI journey.
Earlier this year, Nvidia launched several AI inference platforms to aid developers in building specialized, AI-powered applications. These platforms use Nvidia’s latest inference software, including NVIDIA Ada, NVIDIA Hopper, and NVIDIA Grace Hopper processors. Each platform can tackle AI video, image generation, large language model deployment, and recommender inference. Â
In a recent article by the Motley Fool, Nvidia will reportedly benefit significantly from this oncoming adoption of AI systems. As a company heavily interested in AI with numerous products critical for AI solutions, such as its graphics processing units (GPUs), Nvidia will be one of the front runners of the AI revolution within modern industries. This is mainly due to GPUs' capabilities in terms of AI requirements. Â
AI supercomputers need thousands of GPU arrays to increase performance in quickly solving intense calculations with high-quality graphics and efficiency. For those that can’t build their own supercomputers, Nvidia offers the Nvidia DGX cloud, which allows clients to utilize a specified amount of AI-computing power. Furthermore, its AI training service provides multiple tools to aid an organization’s AI development, from workload management models to chatbots for customer support. Â
PC sales and cloud services expect continued downward trends through Q2 2023 and perhaps the first half of Q3. This will put a damper on AI implementation throughout the year. Still, as companies formulate their AI strategies, sales are expected to pick up around the end of the year when most inventory correction for excess stock finalizes. Â
AI is set to become one of the more beneficial tools that can aid human staff in completing their tasks. One of the best ways to see how greatly your organization can benefit from AI is by using it on a smaller scale before investing in creating your own.
Don’t Plan on Digitalizing? It Will Cost You
The semiconductor industry and the greater electronic component supply chain have a problem. For an industry that supplies manufacturers with cutting-edge technology that brings modern society closer to an almost sci-fi Hollywood-like reality, it is exceedingly traditional. Â
Traditional practices within the electronic components industry mean hundreds of Excel sheets to track market data generated daily. No matter how resistant a company is to embracing digital tools, it is impossible not to possess some form of digitalization. Whether in the shape of email, instant messengers, using e-commerce sites for component procurement, or, yes, Excel. The problem lies in the resistance to further digitalizing and improving existing digital tools. Â
Why is that a problem? There’s a multi-pronged reason why continued digitalization and relying on tradition hurts companies far more than theoretical cost-savings. Â
Data is invaluable. Data provides hard evidence that a product is working, successful, if it needs to be improved, and more. Within the electronic component industry, the supply chain generates oceans of data daily. Some data is less insightful than others, it depends on what function it needs to serve. Â
So, what’s the issue with data? The electronic components industry is filled with a lot of sensitive data. Semiconductors and other electronic components are among the most critical products integral to modern society. They power computers, cars, medical devices, and defense products, among thousands of other things. Therefore, chip designers are very particular about who can access their data. Â
That data is at serious risk if companies fail to digitalize or adopt new technology. Â
Unfortunately, the world has some bad eggs in it. In a recent article by Forbes, writers discussed why technology companies must take digital stewardship seriously. Digital stewardship is the ethical and responsible management of digital assets, including data, privacy, and cybersecurity. For companies that collect vast amounts of data, the cost of being digitally negligent or failing to manage data properly goes beyond cost. Â
This isn’t just a problem for tech companies. The electronic components industry, from OCMs to electronic manufacturing service providers (EMS) and original equipment manufacturers (OEMs) to everyone in between, produces and hosts quite a large amount of data. Failing to protect this information opens organizations up to data breaches, cyberattacks, privacy violations, financial losses, and damaged reputations. Â
IBM studied the effect data breaches had on companies. Data breaches cost $4.35 million globally, but the more considerable loss comes from the damaged trust between customers and a company. Since data breaches can contribute to the loss of intellectual property, legal fees, and regulatory fines, many former clients will cease existing relationships due to diminished trust. If a company fails to protect secured data, whether it just be transactional information from a purchase or intellectual property shared between an original design manufacturer (ODM) and an OCM, the repercussion of having that information accessed by an unwanted third party are enough to destroy any previous partnership. Â
That loss goes far beyond the cost of legal fees and investments in a new cybersecurity system.
Furthermore, the lack of digitalization can quickly put a company in hot water with various government bodies worldwide. The European Union’s General Data Protection Regulation (GDPR) and California’s Consumer Privacy Act (CCPA) are two laws that require companies to protect user data and privacy. Violations can result in significant fines and legal fees. Possessing old digital tools that have not been updated, for example, an old email server, can put even the largest company at risk for data breaches in the form of phishing emails–especially if staff aren’t adequately trained on the risks. Â
Digitalization is an important step for companies, not only for the competitive edge it gives them but for the protection provided to sensitive data. The cost of digital negligence is too great a threat to ignore in this day and age. It’s time to start bringing your organization into the digital age. Â
‍
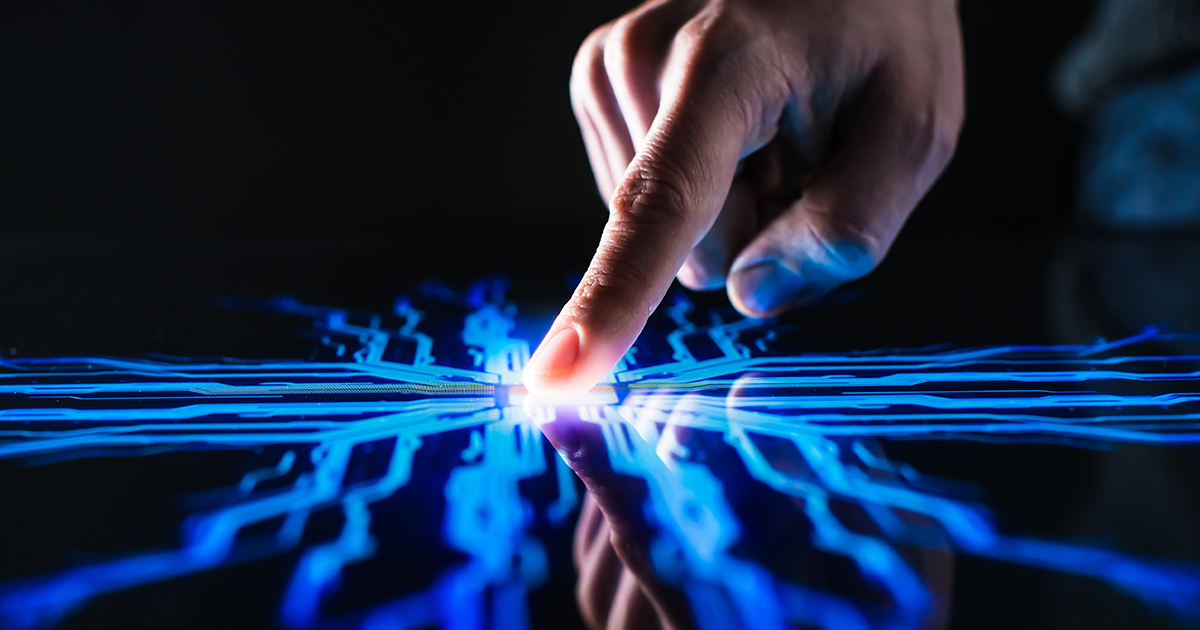
‍If it Ain’t Broke, You Still Might Need to Fix it - May 5, 2023
Is digitalization right for everyone?
That’s a popular phrase in the industry today. Digitalization is a necessity. It is not optional. If you want to stay competitive, you have to digitalize. The semiconductor industry has used traditional operational methods for decades and outlasted numerous shortages. If these methods have worked so well, why must the industry change them? Â
The truth is yes, the semiconductor industry should have left certain operating methods in the past a long time ago. Just because they continued to work long past their “best by date” doesn’t mean they weren’t broken. Like the chips we depend on for our products, there is a short window of time for tools to function at their peak. After a while, and a lot of use, they degrade and decay in performance until they break. Â
The fact of the matter is the semiconductor industry should have digitalized a long time ago. Â
Tradition Fails, New Procurement Processes Take the Lead
There’s an old saying, “If it ain’t broke, don’t fix it.” It’s an American adage often attributed to Thomas Bertram Lance, also known as Bert Lance. Lance was a close adviser to Jimmy Carter during his 1976 campaign and became director of the Office of Management and the Budget (OMB) in Carter's government. Lance was hardly the inventor of the phrase, as it originated long before Lance’s use of it was published in William Safire's Pulitzer Prize-winning article. Â
The phrase is, most often, true. If something works adequately, leave it alone--only fix it if it is broken. Â
But metaphors aren’t something manufacturers can rely on when inaction results in disaster. A method may work well for years, but evolution often trumps adequacy. Â
Before the Covid-19 pandemic, the traditional methods within the semiconductor supply chain worked. If it ain’t broke, don’t fix it. Just-in-time (JIT) scheduling was the primary method of scheduling shipments. Original equipment manufacturers (OEMs), contract manufacturers (CMs), and electronic manufacturing service providers (EMS) would only order from original component manufacturers (OCMs) or electronic component distributors when they needed components. OCMs would determine production capacity for component lines based on previous yearly sales. Market indicators were sometimes taken into account. Collaboration and transparency between manufacturers were almost non-existent. Â
After all, why share designs with an OCM or discuss future stock security if it was never needed? Short-term gains were prioritized over long-term strategies, and when the pandemic hit, the semiconductor supply chain faced one of the worst shortages ever. Â
Semiconductor bottlenecks are born from limited capacity, high demand, and over-ordering. These bottlenecks will likely last throughout 2023, despite continued efforts to mitigate the problem. The chip glut is here, and excess inventory won’t resolve until late 2023 at the earliest. Traditional ordering and even sourcing methods are not cutting it anymore. Many manufacturers are unwilling to adapt right now because time is scarce. Â
Traditional chip sourcing methods are notoriously tedious. Long hours are spent comparing chip offers with numerous calls to different sales representatives. Keeping these methods in place for short-term gains will only delay the inevitable. New methods are more resilient, cost-effective, and time efficient when integrated into a workflow.
It doesn’t even take a lot of time to learn. Â
Many companies are starting to integrate artificial intelligence tools into their organization’s workflows. AI can easily aid companies by quickly collecting and sorting through data to find credible insights for procurement teams. Machine learning algorithms can be customized in their approach to data collection, finding new opportunities traditional sourcing methods can overlook. Organizations that utilize AI are more agile, resilient, and able to routinely run aggressive inventory strategies that benefit both short-term and long-term goals.
Manufacturers that use AI can offer greater transparency to OCMs, allowing them to accurately determine chip production capacity based on a manufacturer’s future needs. Â
One of AI's newest features is the ability to create a digital twin. This AI-powered solution can optimize end-to-end supply arrangements, from the availability of parts to advanced production planning and logistics. AI-powered tools will help optimize the supply chain’s complex web of producers and products while boosting time management—something the semiconductor industry desperately needs. Â
The future shortages the supply chain will face are expected to make the 2020-2022 global shortage look like a walk in the park. Fortifying your supply chain now with AI will prevent the shortage's damage effects from impacting your organization to the same extent again. Â
How to Mitigate the Shortage’s Impact
Recovery is coming, but based on expert forecasts, it won’t be until late 2023 that we can all breathe a sigh of relief. For now, working on building resiliency and supply diversification will help speed-up recovery.
Government incentives and subsidy programs can only do so much, especially when bringing new fabs online takes years. Demand could outpace supply, despite being in a period of excess, with how rapidly digitalization is taking the world by storm. Popular tools like generative AI and the growing Internet of Things (IoT) are expected to feed the demand that brings the semiconductor industry out of the current chip glut. Â
To continue negating the impact of the shortage, you can do a few things to outlast the pain. Â
1. Collaborate with Distributors
Transparency is key. One of the exacerbating problems of the shortage was the traditional method of OCMs and OEMs not working with one another. During the design phase for a product, there should be collaboration between distributors, OCMs, and OEMs regarding what is needed for upcoming production lines. That way, stock can be secured for future capacity, so it is available when a product is ready for market. Â
2. Always Check for Alternates
Sole source components are a dangerous threat that often goes unnoticed or overlooked. The more sole sources your product requires, the more vulnerable it is to shortages. You’ll have a stronger product design if a component has plenty of form-fit-function (FFF) alternates, a diverse lineup of manufacturers, and currently active alternates. You can confirm this through Datalynq’s Multi-Source Availability Score, which takes several factors into account before assigning a component a score based on its availability.
3. Be Ready to Adapt
The semiconductor shortage was one of the more difficult challenges of the recent decade for the semiconductor industry. By adapting to emerging technologies and new methods, manufacturers can create a more robust supply chain that can withstand some of the worst disruptions. Traditional methods acted as kindling, and Covid-19 was the spark. Using digital tools to give supply chain managers and other key decision-makers more visibility over the global state of the supply chain and market.
The shortage’s impact will last for years to come. It was a perfect storm of circumstances that led to the years-long drought of chips, and relying on old methods will only ensure the subsequent shortage is worse. As manufacturers mitigate the damages of the 2020-2022 shortage, it is essential to implement new technology to better prepare for the long-term changes coming to the electronic component industry.
You can explore what AI tools can do for you with Datalynq’s 7-day free trial today. Â
‍

For Those That Don’t Embrace Digitalization, You Will Lose Valuable Data - April 21, 2023 Â
Chip demand is in a slump. While it’s low chipmakers and market experts are urging original equipment manufacturers (OEMs), electronic manufacturing service providers (EMS), and others to invest in transforming their supply chain now. Supply chain managers are working hard to diversify, fortify, and make their supply chains more resilient. The way to accomplish this goal is through digitalization. Â
New artificial intelligence (AI) and machine learning tools are helping organizations across all industries uncover better insights from their data to prepare for future disruptions. While diversifying your supply chain is vital to a supply chain’s strength, strategically planning for possible disruptions through data insights will give companies the competitive edge they need. Â
Moreover, integrating AI into workflows is vital to overcoming future hardships. Â
Chip and Research Giants Give the Same Advice: Digitalize
Digital transformation is what the supply chain needs. The current demand slump has left ten of the top global chip companies down 34% from the $2.9 trillion made in November 2021. Many issues, including rising interest rates, high inflation, lower consumer confidence, and tech-led stock market retreats, have caused the downturn. Pair these economic challenges with more significant disruptions, including Covid-19 lockdowns, seaport congestion, Europe’s energy crisis, and the war in Ukraine, and you get the current state of the semiconductor supply chain. U.S. sanctions on advanced semiconductor technologies in China will likely impact the industry throughout 2023. Â
To combat these problems, original component manufacturers (OCMs) have been cutting costs, reducing their workforce, and lowering production output where possible. These strategic measures are working to mitigate the effects of the shortage-turned-glut and have varying levels of success depending on the niche of the chip market. Memory OCMs, such as Samsung Electronics and SK Hynix, are experiencing some of the worst quarters since 2008-2009 as DRAM and NAND prices fall. Production cuts, scaled-back capacity, and low spending are helping mitigate some of the damage.
But everyone, including chip giant TSMC, faces pitfalls from the current slump.
The industry is working quickly to solve these rising problems, and based on experts’ forecasts, these plans might pay off. The last chip shortage is expected to be resolved by early 2024. Those facing excess inventory challenges should see demand pick up in late Q3 and Q4 of 2023, helping digest the rest of the remaining surplus. All-in-all, the bullwhip from shortage to glut could have been worse. Thankfully, it wasn’t.
However, it could have been a lot better. Â
Industry-leading business consulting firm Deloitte believes that despite the predicted growth in the latter half of the year, the semiconductor industry requires transformation through 2023. According to Deloitte, the U.S. and European Union’s plan to diversify all portions of their supply chains, from fabrication to assembly and testing, will be the first necessary step. Had these steps been taken in the past, many missteps by OCMs and OEMs alike could have been avoided. Â
There are different ways to make a more resilient supply chain. The more diverse your supply chain is, the less vulnerable to shortages, geopolitical tension, and other hurdles. Deloitte’s article states that digital transformation with data-driven supply chain networks is the best way to fortify your supply chain against future disruptions. Â
According to Deloitte’s report, “This is where integrated data platforms, next-generation ERP, planning, and supplier collaboration systems along with artificial intelligence and cognitive technologies are expected to make OSAT processes more efficient and help sense and preemptively plan for future supply chain shocks.” Â
Sharing real-time data and intelligence across the supply chain with digital tools helps OCMs and OEMs address numerous issues from sales and partner organizations and proactively plan for warehouse storage and logistics. It also keeps the entire process transparent, which aids collaboration across the supply chain. Deloitte advises semiconductor supply chain members to adopt industry 4.0 solutions, such as Nvidia’s Omniverse which utilizes digital twins to aid in process visualization. Predictive analytics can assist decision-makers in perfecting mitigation strategies before disruptions occur, preventing the significant losses that OEMs are currently dealing with. Â
Deloitte continued, “In 2023, semiconductor companies need to modernize their ERP systems and integrate diverse data sources such as customer data, manufacturing data, financial and operational data.” Â
AI-Gathered Data Improving Processes Across Industries
It’s hard to stay competitive without artificial intelligence. Today, AI adoption is a necessity, not an option. To keep pace with the ever-changing and more technology-integrated world, AI is needed to help pick up the slack so talented human workers can better aid organizations. Numerous enterprises have embarked on their journey to utilize AI in transforming their businesses. Â
AI is a general term for many technologies, all of which represent some form and degree of digitalization. For some, it is automating assembly line robots, which use AI to perform a predetermined function. For others, it is implementing predictive analytics to help forecast future disruptions based on historical trends or aid assembly robots in the detection of possible defects in products. Â
It’s important to note that the more technology is integrated into the world, the more data is produced. The vast quantities of data generated daily can no longer be analyzed swiftly and efficiently by human staff. The best way to organize and understand it is best accomplished through AI aiding workers by quickly presenting relevant data that supports an organization’s objective through big data analysis. Other AI software platforms should function similarly.
Each tool should serve a specific function that supports the overall business goal. One such tool, ChatGPT, or generative AI, is new on the market. Business leaders are now seeking ways to integrate this latest tool into their workflows to maximize benefits. To determine whether a new piece of software will benefit your company is to first assess the existing data strategy and how it is maintained. If an organization is to implement the latest and greatest tool without a proper understanding or strategy in place, organizations can quickly be bogged down and inflexible. Â
Supply chain managers should implement strategic data sourcing, which requires a defined objective, decision, and outcome regarding collected data. AI can be utilized in this position to seek out internal and external data, like data scientists, to find relevant, appropriate, of high-quality, and permissible to use. Better yet, AI aids data scientists and other staff in this task by helping identify high-quality data quickly through its given rule set. It forces data management teams to set transparent objectives and rules to determine necessary data. Once trained, AI can quickly sort through the mountains of real-time data finding pieces that fit the predetermined set of rules.
Once completed, data gathered by AI helps define and drive digital transformation. Datalynq can help digitalize your supply chain through predictive analytics, a solution that uses AI to forecast market trends, prices, and possible disruptions through real-time market data. You can get started with a 7-day free trial to see how Datalynq can help you today. Â
‍
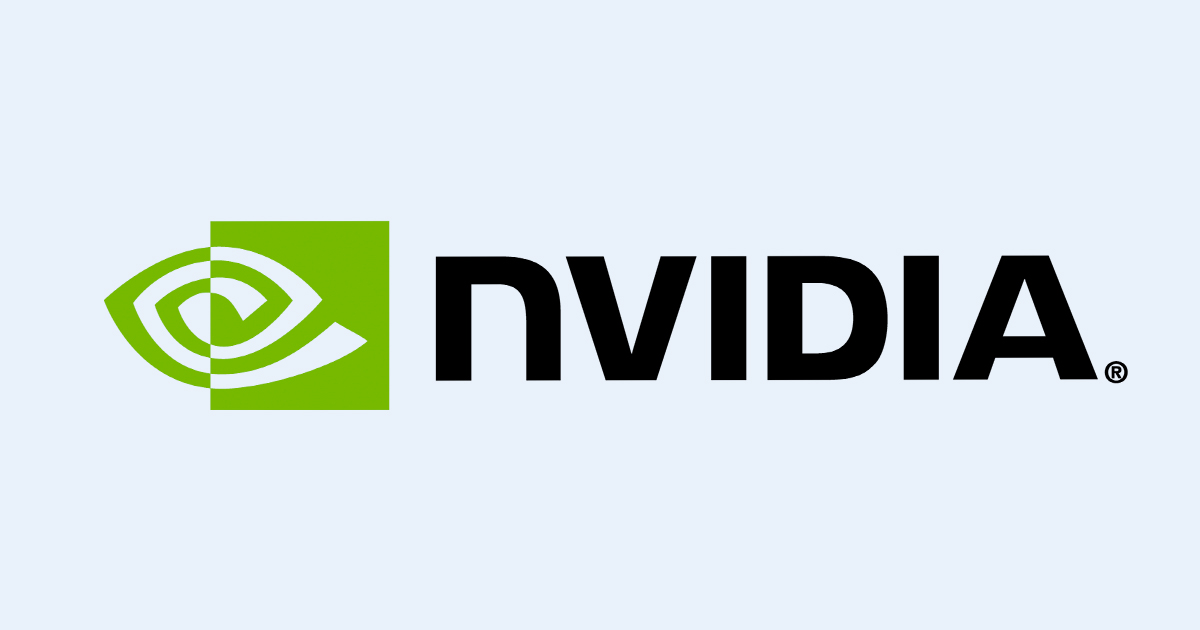
New Day, New Tech - April 7th, 2023
2023 is quickly becoming the year of new technology. After several years of the chip shortage, companies are making up for the lost time. With intelligent tech, the number of chips needed to support their efficiency is increasing with every advancement. Now that advanced chips are more available, due to consumer demand slowing from inflation costs, artificial intelligence is rising in popularity and application. Â
Next-Generation IoT Prioritizes Connectivity
Let’s begin with a simple fact. Technology today makes it hard to imagine a world where we aren’t connected. Connectivity is important to us, whether between people, organizations, or devices. It should come as no surprise that as technology develops in the modern age, the focus on connectivity and how a device connects users with other devices are prioritized. Smart, interconnected devices are intertwined with daily life as it helps make mundane tasks easier. It allows users and others to benefit from connected devices more productively and efficiently. Â
Connectivity is an inherent piece of smart technology. Smart technology is “a technology which uses big data analysis, machine learning, and artificial intelligence to provide cognitive awareness to the objects which were in the past considered inanimate.” AI and other learning algorithms within smart technology usually involve connection with, at minimum, another platform capable of data collection or hosting. As it learns and interacts with the data, it communicates results to users or other devices following predetermined actions programmed within it based on a dataset pattern. Â
The Internet of Things (IoT), a network whose purpose is to connect numerous devices and their data, prioritizes connectivity as it develops. With each technical advance in lower power chips, AI, and machine learning, new uses for IoT become known. Their advanced connectivity allows for better and faster data sharing. In a recent report by McKinsey and Company, it’s expected that by 2030 IoT products and services will create between “$5.5 trillion and $12.6 trillion in value.” While today’s IoT is still somewhat fragmented, thanks to its variety of tech ecosystems, IP, and standards, insights from today’s challenges can uncover new solutions. Â
As a result, IoT solutions are being utilized in numerous industries that rely on quick and efficient data sharing. Manufacturing, healthcare, and transportation will be some of the key markets expected to benefit immensely from improved networks that lead to connectivity between applications where none previously existed. By 2030, McKinsey expects factories and medical sectors to account for 36% to 40% of value. Â
Rob Conant, vice president of software ecosystems at Infineon, told McKinsey and Company that IoT applications are spreading from industry to industry and into more diverse businesses. “Connectivity is extending into more and more applications,” Conant said. “Pool pumps are becoming connected, light bulbs are becoming connected, even furniture is becoming connected. So, all of a sudden, companies that were not traditionally tech companies are becoming tech companies because of the value propositions they can deliver with IoT. That’s a huge transformation in those businesses.” Â
According to McKinsey, the best way to improve IoT solutions faster is to enhance collaboration between software, hardware, and chip manufacturers alongside IoT product makers. Alignment of goals through each member of the production chain in bringing these solutions to fruition will lead to innovation at a faster pace. If the focus on new IoT products is to improve connectivity between devices and users, it should also be the priority of those who make them. Â
Collaboration and transparency in all sectors of a supply chain, from design to market, can give the organizations working together a competitive edge that jettisons them far beyond their competition.
Nvidia Launches AI Interfaces to Help Integration in Workflows
The future is here. 2023 is quickly becoming the year of AI. OpenAI’s ChatGPT, Google’s Bing, and Microsoft’s Bard kicked off 2023, and the train of AI applications is only just getting started. On March 21st, Nvidia launched a series of inference platforms for large language models and generative AI workloads. As a result of AI rapidly growing in popularity, Nvidia’s latest inference platforms are optimized to aid developers in quickly building specialized, AI-powered applications.
Each inference platform has been combined with Nvidia’s latest inference software, including NVIDIA Ada, NVIDIA Hopper, and NVIDIA Grace Hopper processors, which utilize NVIDIA L4 Tensor Core GPU and H100 NVL GPU. The four platforms are equipped to tackle AI video, image generation, large language model deployment, and recommender inference.
With AI’s use rising in prominence among numerous industries, and only poised to continue its vast reach, Nvidia CEO Jensen Huang knows it is only limited by imagination. “The rise of generative AI requires more powerful inference computing platforms,” Huang said in Nvidia’s product announcement. “The number of applications for generative AI is infinite, limited only by human imagination. Arming developers with the most powerful and flexible inference computing platform will accelerate the creation of new services that will improve our lives in ways not yet imaginable.” Â
With the steady increase and utilization of AI in numerous industries, Nvidia is attempting to position itself as the go-to partner for AI development. Partnering with Oracle Cloud, Microsoft Azure, and Google Cloud, Nvidia is making its AI supercomputers available as a cloud service through Nvidia DGX Cloud. The goal is to help enterprises train models for generative AI and other AI applications based on the quick rise and experimental implementation of other generative AIs, like ChatGPT. Â
This announcement and new products come on the heels of Nvidia’s Omniverse improvements. Nvidia’s Omniverse is an industrial metaverse that provides “digital twins” of processes or products to aid in predicting how it would perform throughout time. Nvidia’s new powerful GPUs help better visualize the techniques. Â
Nvidia’s tools are a small indication of the greater change within the modern industry. AI utilization in any form, whether generative or others, significantly increases organizational innovation, productivity, and efficiency. The further they improve, the greater they aid workflows and help decision-makers strategize based on results derived from the seas of data generated. Implementing AI into your company will soon become necessary, not an option, with how greatly AI can improve certain parts of an organization.
The best way to begin digitalizing your business through AI is to incorporate a tool that provides multiple features for various solutions that handle tedious tasks. Datalynq is equipped with market intelligence scores that water down large amounts of data into a 1-to-5 scoring system on component availability, price, risk, and more. The predictive analytics and alerts solution warns users of upcoming disruptions long in advance, giving organizations time to prepare and document everything through Datalynq’s case management system. At the same time, the staff focuses on larger, complicated projects. Â
Want to increase innovation and productivity in your company’s workflows? Try out Datalynq’s 7-day free trial! Gain better insights and visibility throughout your supply chain today.
‍

‍Data Analytics and AI, How Components Are Changing Everything from Healthcare to Manufacturing - March 24, 2023
Digitalizing your organization is for more than just tech industries. Artificial intelligence (AI), machine learning, big data, predictive analytics, and more are changing how organizations operate in the 21st century. After the 2020-2022 chip shortage, development in AI and its capabilities has resumed improving rapidly. Each improvement brings better benefits through enhanced accuracy and faster results. Â
Healthcare, notorious for its complex and niche fields, benefits immensely from the inclusion and aid of AI. Predictive analytics and AI, in general, partnered with human staff, through studies have proven how efficiently work can be done when operating in tandem.
Predictive Analytics is a Great Tool for Manufacturing and Precision Medicine
Technology truly is a beautiful thing. Each innovation benefits everyone, from an organization's production lines right down to the consumer. Every industry saves time and cost while increasing efficiency with the aid of digital tools. Any business sector, from retail to healthcare, can operate more smoothly with the aid of AI and the many tasks it can accomplish Â
A rising star within AI is predictive analytics. While it is not necessarily a new feature, its aid in numerous industries has recently risen in popularity and further implementation. That includes healthcare. Â
While AI, in general, is not new to medicine, predictive analytics, a great tool for manufacturers, is now on the rise. Predictive analytics identifies patterns and trends through data analysis, thereby providing alerts and possible solutions to human decision-makers. The biggest boon of predictive analytics in the semiconductor manufacturing supply chain is sorting through mountains of data quickly and accurately. The semiconductor supply chain, a large and usually complicated chain of original chip manufacturers (OCMs), original equipment manufacturers (OEMs), distributors, clients, and more, produces proverbial oceans of data daily by each member within it. Â
Predictive analytics is not limited to supply chain forecast management. It can also be utilized to predict when robotic assembly tools will go down for maintenance. This ensures that operations can be accurately scheduled around that time frame. Predictive analytics in chip manufacturing can also quickly forewarn manufacturers of possible risks in the form of sole source components. Sole source components face higher prices, longer lead times, and obsolescence, as there are no form-fit-function (FFF) alternates to replace them in the future. Â
With the boon predictive analytics provides to manufacturing, is it a surprise it has also become an excellent medical assistant? Predictive analytics in precision medicine can provide personalized treatment plans and associated risks of a patient’s genetic history, lifestyle, and environmental data. It can alert providers to specific conditions and diseases, such as cancer or heart disease, for patients long before symptoms appear. Successfully aiding in proactively treating patients long before malignant tumors or illnesses strike. Does that sound familiar? Â
Healthcare is just as, if not more complex, than the semiconductor supply chain. The amount of data the program must sort through to accurately predict future events and outcomes is immense and only continues to grow. Luckily, most developments within AI are happening at the same rapid pace that both medicine and technology have set, making it sensible to integrate predictive analytics into an organization so it can learn these advancements as they’re developed. Â
AI is Being Utilized to Detect Cancer
Pattern recognition within AI has been a function steadily perfected over decades. It’s a vital component of most AI systems, as machines can easily identify patterns in data. Once it recognizes a pattern, AI can make decisions or predictions using specific algorithms. This crucial component of AI can be utilized in many fields and industries. Â
Radiology is not a new industry for AI. In 2018 in an article published by the National Institutes of Health, a team of doctors examined the relationship between AI and radiology. Specifically, the authors discussed the general understanding of AI and how it can be applied to image-based tasks like radiology. Recent advances in AI-led doctors realize that deep learning algorithms could lead AI models to exceed human performance and reasoning with complex tasks, as current AI models can already surpass human performance in narrow task-specific areas. In some regions of medicine, such as radiology, the early detection of cancer can make a big difference in the patient's mortality. Â
In the 2018 article, the authors noted that using AI in mammography, a particularly challenging area to interpret expertly, could help identify and characterize microcalcifications in tissue. In 2023, AI is being used to help successfully identify breast cancer in mammogram screenings. This AI is utilizing an advanced form of pattern recognition to assist radiologists in analyzing the images’ details. Â
The AI used is called computer-assisted detection (CAD). Studies have shown that CAD helps review images, assess breast density, and flag high-risk mammograms that radiologists might have missed. It also flags technologists for mammograms that need to be redone. A study published last year found that CAD was just as effective, if not more so, than a human radiologist in a faster period. Â
One doctor who spoke with the New York Times stated, "AI systems could help prevent human error caused by fatigue, as human radiologists could miss life-threatening cancer in a scan while working long hours.” While doctors and AI development teams agree that AI can never replace doctors, AI-human teams reduce the workload of radiologists by having an automated system quickly and accurately provide a second opinion. Â
This partnership is true for all industries that incorporate AI into their organization. The goal of utilizing AI shouldn’t be to replace human staff but to aid them in accomplishing goals faster and more accurately. Continued advances in AI show that it is versatile and can be trained to perform numerous tasks, from cancer detection to even product defects. Implementing AI into your organization can be a time and cost-effective strategy.
‍

Why Case Management is Necessary for Any Design Strategy- March 10, 2023
The digital tools we use are only getting smarter. Machine learning is quickly becoming part of organization workflows, but it shouldn’t stop there. Machine learning can provide a strong foundation for several manufacturing operations, decreasing costs while improving production line efficiency. Â
Component case management is an easily overlooked strategic aspect in the electronic component industry. For many original equipment manufacturers (OEMs), the only element given any strategic overview is sourcing and when to schedule orders. But none of that matters if you aren’t investing in a tool that can aid you in successfully managing components necessary for your products from start to finish. Â
Component case management should be done during the initial design phase so that risks are discovered and mitigated long before they become a problem. Â
Why You Need Component Case Management
The traditional reactivity method in response to component obsolescence, shortages, and other disruptions is no longer applicable in a post-pandemic supply chain. Proactivity concerning component management is necessary for the supply chain of today. What was solved through simple communications with suppliers is no longer applicable in the complex, global supply chain that is continuing to diversify. Strategizing for component risk factors must be done as early as the design phase. Â
Otherwise, one might lose costs and time reacting to problems through damage control, much like the automotive industry had to during the pandemic. Â
Manufacturing case management is a dynamic process that assesses, plans, implements, coordinates, monitors, and evaluates to improve outcomes, experiences, and value. In the case of electronic component manufacturing, case management is actively pre-planning for scenarios, the expected downtime, and the cost for the preplanned response. That could be anything from component obsolescence impacting products and planning a last-time-buy (LTB) before a manufacturer ceases production to complete product redesign around a component. Â
Component case management is usually used to plan a documented strategy to mitigate and resolve the issue in preparation for component obsolescence. However, OEMs can use case management for many problems beyond obsolescence management. Documenting plans that coordinate strategies to resolve these issues lead to better efficiency in resolving them. Likewise, for many defense OEMs, these documents on case management are required. Â
Datalynq offers case management for users that aids in identifying issues you may have with parts, opening a case on these problems, and taking action to resolve them. When you open a case in Datalynq, you can add the expected impact date, the case status, the government case number if acquired, the number of days production will be impacted, the impact rate of logistics and repairs, and more. Once you’ve initiated a case within Datalynq, all your pertinent case information is documented in an audit trail.
You can also add information for potential resolutions, their cost, the summary of the mitigation plan, and even the confidence of how this mitigation strategy is expected to work. These documents provide full transparency to government agencies, like the Department of Defense (DoD), which require it. For other OEMs, visibility into product case management helps smooth the resolution process and can be shared easily with the necessary departments to implement such a significant change. Â
If you want to see how easily Datalynq’s case management system can improve manufacturing processes effectively, Datalynq’s 7-day free trial lets you take control.
Machine Learning Transforms Manufacturing
As part of the greater umbrella of artificial intelligence (AI), machine learning is “the use and development of computer systems that can learn and adapt without following explicit instructions by using algorithms and statistical models to analyze and draw inferences from patterns in data.” Machine learning is an intelligent program that learns through studying data to predict, detect, and provide analyses through its algorithm. Â
Machine learning usually comes to mind when people think of AI, as it is often utilized in natural language processing (NLP) chatbots to better imitate human speech. The popular ChatGPT possesses some machine learning in its program as its algorithms are pre-trained by data. This pre-training then aids in generating text, whether small chat box responses or entire articles, close to human speech. Â
Beyond chatbots, machine learning is a rapidly expanding field thanks to its endless potential in many applications. Any industry can utilize machine learning, from retail to healthcare. It is particularly helpful in manufacturing applications. OEMs can use machine learning within manufacturing for quality control through defect detection, automation of repetitive work in production lines, and customization of products. Â
Utilizing training algorithms to help identify product defects from images and other data sources can help reduce the cost of quality control while improving inspection accuracy. Along those same lines, machine learning can be paired with another subset of AI called predictive analytics. Together these programs can detect, predict, and forecast when automated production lines need maintenance and how long they’ll be nonoperational. Â
The repetitive nature of production lines makes machine learning the perfect tool for automation. Assembly line robots can run off machine learning algorithms trained to perform many tasks, from welding to part fabrication. Automation with machine learning cuts down on operational costs while increasing efficiency. Automating production lines also frees human staff from tedious but necessary simple tasks so they can put their time and attention into more innovative projects. Â
Customizing products with automated production lines trained through machine learning is far easier. Time spent customizing products through manual labor and individualized assembly lines would no longer be necessary. Machine learning can abide by the data that comprises these custom designs without incurring additional costs of individualized production lines. It simply needs to be told when and how to do it before it starts creating. Â
Machine learning technologies will continue to be implemented far into the future beyond the small forays of ChatGPT into general workflows. With how competitive the current global supply chain is, reducing operating costs and time to manufacture products will give companies an edge many hesitate to take advantage. It’s time to get started. Â
Â
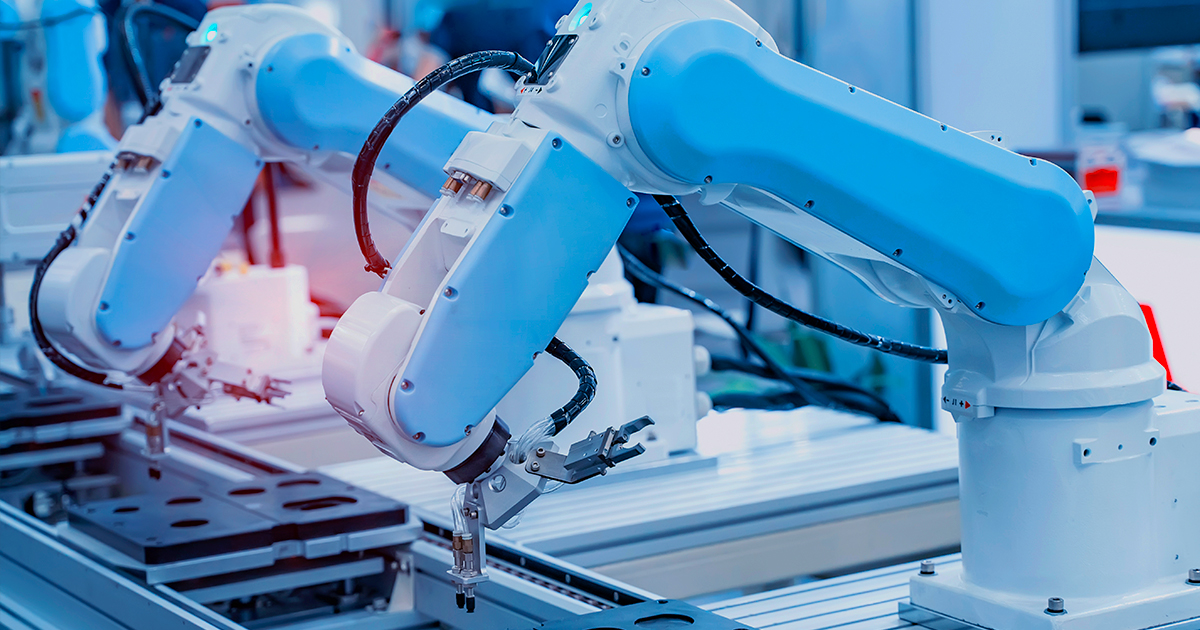
Data is Transforming the Semiconductor Supply Chain - February 24, 2023
The world is becoming more connected. As it does the amount of data that these connections produce grows too. The global supply chain produces a significant amount of data every year but still needs more visibility for industry members to be able to glean all the critical insights within it. To manage this information, data analytics and artificial intelligence are vital to collect important supply chain information. Â
As data-driven tools become more advanced, so does the information it delivers to users. To stay competitive and keep further disruptions from impacting the world’s supply chain, original equipment manufacturers (OEMs) must adopt these tools. If not, manufacturers might miss some important red flags that warn of future disruptions, like those brought on by the pandemic.
Semiconductor Supply Chain Issues Are Negated by Data Analytics and AI
It should be no surprise that data-driven analytics and artificial intelligence (AI), among other digital tools, help mitigate supply chain disruptions. After experiencing unexpected events derailing plans over the last several years, supply chain managers are eager to keep history from repeating. Rohit Tandon, the managing director and global AI and analytics services leader at Deloitte, explained the only way to prevent future disruptions is to know what they are and plan accordingly.
“The Covid-19 pandemic vividly illustrated unexpected events' impact on global supply chains. However, AI can help the world avoid similar disruptions in the future.” Tandon said, “AI can predict various unexpected events, such as weather conditions, transportation bottlenecks, and labor strikes, helping anticipate problems and reroute shipments around them.” Â
The supply chain is a complicated beast that needs dedicated monitoring, which today only a program can sufficiently manage. AI and other machine learning algorithms accomplish this by crunching through massive amounts of data generated daily by the electronic component supply chain. The data, far too much for a team to sort through and analyze as quickly as AI can, is growing in specificity and amount each year. Â
This increased transparency can lead to improvements in operating efficiency, which, in turn, boosts working capital management with fewer supply disruptions. “Manufacturers that are using AI for visibility,” said Tandon. “Can better respond to potential disruptions to avoid delays and pivot if needed…organizations can leverage data analytics for deeper insights across the supply chain.”
Even better, these tools are designed to improve demand prediction and support data sharing with customers and partners. So, everyone benefits from the insights. The increased transparency also helps fortify supply chain resilience and build trust in the output of analytics and AI processes. As these tools develop, it becomes easier to identify trends and patterns to guide customers through market conditions years into the future. It can point out design risks when using specific components if they are a sole source, EOL preventing costly future redesigns or increased shortage risk. Â
The most crucial factor to consider is finding a tool that provides the most accurate data so that when information is shared, it helps, not hinders. The most capable market intelligence tool that combines real-time market data and predictive analytics with other management algorithms is Datalynq. Â
Cyber-Manufacturing Improves the Global Supply Chain
The CHIPS and Science Act are making OCMs approach manufacturing in the U.S. a little differently. The U.S. needs more skilled labor to become a chip-manufacturing powerhouse. However, there are two solutions to that problem.
At the start of 2023, tech giants in Silicon Valley began laying off staff in massive waves, thanks to consumer demand slowdown. As a result, the talent pool of skilled candidates increased. This influx of experienced labor is not as large as the talent pools inside India and Vietnam, but their experience with Silicon Valley’s tech leaders can kill two birds with one stone. First, they can help support the new facilities in research, development, and manufacturing with their technical expertise. Secondly, they have the expertise to properly manage a cyber-manufacturing line efficiently.
What is cyber-manufacturing? It refers to a modern manufacturing system that offers an information-transparent environment to facilitate asset management, provide reconfigurability, and maintain productivity. The real-world application of cyber-manufacturing is utilizing automation, artificial intelligence (AI), the Internet of Things (IoT), and other data-driven analytics to provide transparency. Most industry experts believe that the modern manufacturing model further embraces automation tools for production lines to save on labor expenses. The future workforce with more competitive OEMs will be tech-savvy academics that utilize predictive analytics to forecast downtime and maintenance on machines. Â
Covid-19 showed how dangerous unplanned and unexpected failures complicated the global supply chain. Further developments in cloud and quantum computing through AI and machine learning are expected to lead to additional visibility of production line health. Vulnerabilities will be easier to spot, and the costs to maintain this type of production will be lower in the long run. Â
When more manufacturers embrace cyber-manufacturing, the greater resilience of the world supply chain develops. The less unpredictable events occur on automation lines, the less likely it will impact other manufacturers and suppliers further down the chain. The best way to get started is through digital tools that support case management for design components. Datalynq does this task effectively and only grows in accuracy.
‍

Data Can Manage Your Stock Better, But Sole Sources Can Undo That Step - February 10, 2023
Too much stock, too little stock, and no stock because the sole source manufacturer cannot meet demand or is otherwise impacted. Many of us have dealt with that over the past several years. Unexpected demand highs and lows paired with disruptions turned buying stock into a gamble. No matter what original chip manufacturers (OCMs) and original equipment manufacturers (OEMs) tried, everyone wound up with the short end of the stick.
But what else can you do? In the face of unpredictable consumer demand and weather, how can you accurately predict what will be short one day and excessive the next? Fortunately, there are digital tools available that can do all that and more. Â
Predictive Analytics is the Key to Prevent Backlogs and Excess
Over the last few years, manufacturers have experienced one of two things—inventory backlogs or excess stock. Many of us have been without supply-demand stability for a while, and we might be in for one or two more years. Why?
At the pandemic's start, automotive original equipment manufacturers (OEMs) cut chip orders. In response, original chip manufacturers (OCMs) cut capacity for automotive chips. Chip capacity is determined by order amount, and with no orders, there was no reason to keep capacity for automotive chips. Only automotive demand spiked long before automotive OEMs thought it would and no OCMs had the chips to meet the growing demand. Â
Since the start of the pandemic, many personal electronics and other white goods OEMs continued to make large orders, or double order products, from eager consumers. OCMs, as a result, increased chip capacity for them. Then in July 2022, demand quickly dropped as recession fears mounted. Â
OEMs tried to cancel orders, but it didn’t work. Many OEMs are left with six months of stock and no product demand. Â
And there are many other instances of lacking data, market visibility, and historical trend analysis that led to frustration from either backlogs or excess stock. The result is lost costs and time, production stalls, delayed product development, and more. It is imperative to prevent either scenario from taking place. How are OEMs expected to stay on top of market trends and more if disruptions make time scarcer than the components themselves? Â
Predictive analytics is the solution. Â
Predictive analytics is exactly what you think. It predicts customer demand and forecasts future market trends. Rather than manually tracking inventory data, predictive analytics takes mountains of real-time data to forecast demand according to market trends, weather patterns, and other variables to determine future demand needs. Â
Datalynq, a market intelligence tool that utilizes predictive analytics, uses component sales data from a global, open-market and machine learning to deliver insights on future part availability. You’ll never be caught off-guard when making strategic orders months or years out. It’s time to stop being caught by surprise when Datalynq is ready to use. Â
How the Shortage Reminded Us of the Danger of Sole Sources
The 2020-2022 semiconductor shortage was a rough ride. The shortage’s easement has finally arrived, but there are still areas of chip scarcity that could continue for a few more years–especially with automotive components. The effects are extensive and could last for years into the future. While there are ways, we can mitigate such devastating effects through predictive analytics and market intelligence, these methods will be worthless if one simple step isn’t taken. Â
It’s preventing sole source components in your product designs. Â
What are sole sources? A sole source for a component entails it has no form-fit-function (FFF) alternates and is not manufactured by more than one OEM. Sole source components are an inherently dangerous design risk. The reason is simple and one that the shortage proved over and over throughout its course. There is no backup if bad weather, logistics issues, geopolitical strife, raw material shortages, and more impact the supply chain for sole source components. A manufacturer must wait for the stock to become available again or, if lucky, buy excess inventory from another. Â
Once a sole source enters obsolescence, manufacturers have no choice but to redesign. As there usually are no alternate components that mimic the form, fit, and function well enough, manufacturers need more wiggle room. Redesigns themselves are time-consuming and costly during normal market conditions. In a period of shortage, those prices shoot up.
Sole source components are usually far costlier than multi-source components. As sole source components are unique and scarce by nature, their prices are generally far higher than non-sole source components. While predictive analytics can warn manufacturers of upcoming shortages and price trends rising, it doesn’t do much to ease either challenge for sole sourced parts.
Another misstep manufacturers can make when trying to avoid sole source components in their BOMs is not identifying who manufactures the alternates and where they’re based. While a component might have existing alternates, they could all be produced by the same manufacturer. Despite the alternates making it a little easier to secure stock, if the OCM is impacted, OEMs, contract manufacturers (CMs), and others still wind up with no stock. Â
Another concern when sourcing components and preventing sole source is failing to identify whether alternate components are active. If the alternates for a component are all inactive, then it’s no better than having no existing alternates.
The more resilient your BOM is the easier it will be to mitigate minor and major supply chain disruptions. You need a tool to measure your BOM and design risk for sole source components. Â
Datalynq’s Multi-Source Availability Risk Score uses real-time data to assess numerous attributes, from the number of unique manufacturers to active FFF and DIR alternates. It then breaks the information into a brief, easy-to-understand window for quick, decisive decisions. Take back control of your product design now to prevent tomorrow’s problems with help from Datalynq.
‍

Component Obsolescence is About to Make Waves - January 27, 2022
The market is in a precarious position. The shortage is easing but not over, and a chip surplus is beginning but only for some. Outside of chip excess and scarcity, the global supply chain is still in an odd flux. Automakers want the capacity for legacy nodes to increase while chipmakers cut what is no longer in demand. Â
What that means is component obsolescence, and a lot of it is just around the corner.
Germany Takes Steps to Decrease Obsolescence Risks During Future Disruptions
In an article by Supply Chain Connect in 2018, two years before the 2020-2022 shortage, industry leaders described obsolescence as a supply chain threat. The reason is simple to understand. Managing component obsolescence takes time, money, and logistics to handle. Obsolescence in electronic components is a persistent and never-ending challenge. Â
It is not a matter of “if” obsolescence will occur. It is a matter of when.
Component obsolescence is an inherent complication with only a few solutions. One can procure a sizeable last-time purchase of components as they enter the end-of-life (EOL) stage. Another solution requires finding form-fit-function (FFF) alternates to replace the obsolete component. Sometimes last-time purchases or FFF alternates are unavailable, especially if the components are from a sole supplier or used in specific industry devices. That requires a redesign.
It is a lot easier to redesign a phone than it is a defibrillator. The latter requires time-consuming and costly testing to ensure it follows stringent regulations. Medical devices, among other products, must have every part approved. Even replacing a component with an FFF alternate is costly. Â
Obsolescence becomes much more complicated when the global supply chain is in a period of shortage or excess. If mitigating a natural part of a component’s lifespan is considered a supply chain threat far before the impact of the shortage occurs, it is pertinent to take proper steps to minimize the effects. To aid in preventing such far-reaching consequences, Germany is fortifying its resilience against such profound risks. Â
“The German Electronics Design and Manufacturing Association (FED) and the Component Group Deutschland (COGD) signed a cooperation agreement at Electronica, Munich,” Evertiq reported. “The agreement ensures coordinated representation of interest with political decision-makers and networking in research and development.”
Further, both organizations plan to develop training courses and lectures to better inform others of proactive and strategic obsolescence management. The goal is to bring attention to obsolescence by creating long-term strategies.
“Efficient, proactive obsolescence management starts in the design. If risky components or materials are used at this early stage, the subsequent effort required to correct the problem is all the greater.” Dr. Wolfgang Heinbach, an honorary chairman of the COGD board, said this on COGD’s collaboration with FED and the importance of obsolescence management. Â
Current geopolitical uncertainties and other imponderables will make the effects of obsolescence risks more significant and frequent. These challenges apply beyond electronic components and their raw materials and software products. Â
“We have now reached a point where obsolescence can pose a significant risk not only to individual companies,” Dr. Heinbach warned, “but also, worst case, to entire sections of our national economy.”
2023 Obsolescence Outlook, What Are the Challenges?
The shortage might be easing, but the supply-demand balance is not here. With automotive components still scarce and advanced chips piling up, the global supply chain is still a year away from stabilization. As excess stock builds, manufacturers across the supply chain are moving into the next stage.
That stage is inventory correction. As consumer demand deteriorates, so does the need for chips. Increased capacity for specific chips will be limited without demand funding as original component manufacturers (OCMs) cut back production. This new limited capacity brings a worrying fact to the table. Â
A lot of components are about to enter obsolescence. Â
The semiconductor market worldwide lost $240 billion in value last year. Excess stock is forecasted to be a problem throughout 2023 until late Q3 and possibly early Q4. In late 2022, TSMC had ten of its top clients cancel orders. These orders were supposed to get them through 2023 alone when product demand was still high. Many original equipment manufacturers (OEMs) are now stuck with six months’ stockpiles. Â
Now it isn’t. In the face of production stalls and another year of revenue losses, many OCMs are digesting what inventory they can. OCMs will cut capacity to avoid losses. With this limited capacity and no demand, plenty of advanced chips will become obsolete, possibly before product demand picks back up. Â
Innovation doesn’t stop. TSMC, as an example, has already begun production of its 3nm nodes. Samsung’s plan puts 1.4nm into total production by 2027, beginning with 3nm in 2024 and 2nm in 2025. TSMC is considering price cuts on their 3nm during this inflationary period to attract more buyers and increase capacity. Other components enter obsolescence to make room.
Overcoming obsolescence will be complicated by the current factors impacting the supply chain, including shortages, excess inventory, macroeconomic pressure, inflation, and more. Being proactive now by finding FFF alternates, sourcing another supply elsewhere, and even redesigning existing projects if necessary while early enough, will make the difference. Datalynq will help mitigate obsolescence’s effects starting now.
‍

Welcome to 2023 From Datalynq! - January 13, 2023 Â
Welcome to the new year! We have many exciting adventures planned for 2023, starting with this new blog dedicated to market news. This blog will bring you the latest market news, obsolescence management, component lead times, and more by updating biweekly.
As a digital market tool, Datalynq condenses large amounts of data across the supply chain and millions of parts into one easy window. After the twists and turns over the past year, it’s hard to keep track of if you are used to the traditional way of storing information on spreadsheets. That’s why we’re ready to give you a crash course in why digitalization and digital tools will be key in coming out of 2023 on top. Â
Datalynq and Market News
The supply chain suffered setbacks over the last few years. Now, it’s in a tumultuous state of extremes. The automotive industry can’t get enough parts to keep production lines open. Consumer electronics manufacturers are up to their necks in components they no longer need. While no significant disruptions are affecting the global supply chain now, it would only take one to throw this delicate balance into chaos.
That’s where Datalynq comes in. It replaces traditional spreadsheets with information that’s easy to digest. Datalynq’s market intelligence monitors fluctuations in the electronic components industry. It makes obsolescence management easy, lead time tracking a breeze, and preparing for future component risks. Excel spreadsheets and hours of phone tag chases are no longer needed.
Datalynq accomplishes this with its Market Availability Score rating system that supplies a comprehensive view of component obtainability based on information compiled from historical trends, suppliers, and market forecasts. The Design Risk Score rating system also uses insights from supply data, lead times, and product lifecycles to determine the risks of designing a part in-house. These ratings are then scored from 1-to-5 for easy legibility, with five being the worst and one the best. Â
Technical data for components are at your fingertips and readily available on Datalynq, so you can ensure the part is the best fit for any project. Along with pricing and inventory trends, users will always make the most informed choice with Datalynq’s aid. It doesn’t take long to learn how to use it, either. With straightforward analysis, users only need a few minutes to understand how to best optimize their projects and supply chain. Â
Digitalization is the Way to Go
It’s time to invest in digitalization. Manufacturers like Qualcomm are encouraging OEMs, CMs, ODMs, and EMS providers to invest in digital tools as the new year begins. Whether through automation, artificial intelligence (AI), machine learning, or other digital tools, the theme of 2023 should be expanding your digital footprint. Why? The more you invest in implementing these tools, the more you’re bound to benefit. Â
Digital tools make it easier to prevent production stalls, navigate shortages, and strategize for component obsolescence. Machine learning can help companies with their algorithms called predictive analysis. These algorithms can predict disruptions by analyzing historical trends and market data.That amount of data would be far too time-consuming and tedious for human analysts to predict as accurately in the short time it takes machine learning tools. Â
AI can give users greater supply chain visibility, which many lacked in early 2020 when decreasing consumer demand at the beginning of the pandemic led to canceled orders en masse. This visibility helps users make more informed demands and keeps miscommunication from happening as it did in early 2020. It took a few months for that demand to turn and skyrocket sharply, leading to the 2020-2022 chip shortage. Â
Automation of processes is imperative beyond production lines. Automating processes save time and give it back to staff to focus on more critical task. OCMs like Qualcomm are taking steps to automate semiconductor manufacturing. As the process is labor-intensive, automation is necessary to avoid production stalls if another pandemic occurs–which previously kept staff home and unable to continue producing chips. Â
These are just a few of the ways to begin your digital journey. It’s not a one-size-fits-all solution, as different tools are more beneficial to some than others. The industry is becoming far more reliant on data, especially within the EV industry. To create better EVs, quickly analyzing mountains of data to design better features will be imperative to stand apart from the competition. This is also true for consumer electronics, medical, industrial, and other industries. Â
While that may sound like a monumental task to begin the transformation, there are easy and quick tools to get you started. Datalynq is one of those tools.